Instructions
Objective
Write a Python homework program to implement SVM classification.
Requirements and Specifications
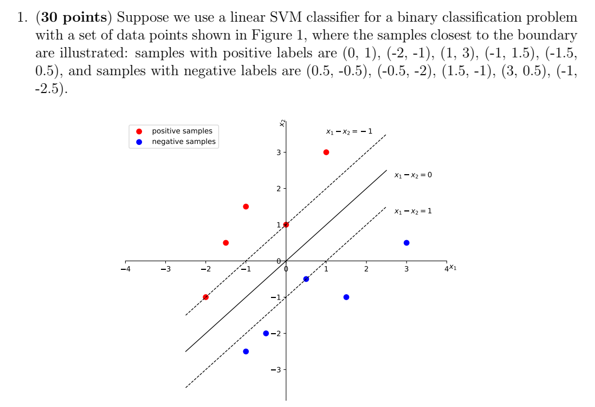
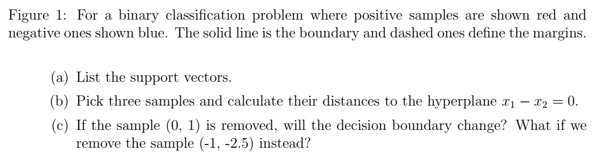
Source Code
import numpy as np
class Tree_node:
"""
Data structure for nodes in the decision-tree
"""
def __init__(self,):
self.is_leaf = False # whether or not the current node is a leaf node
self.feature = None # index of the selected feature (for non-leaf node)
self.label = -1 # class label (for leaf node)
self.left_child = None # left child node
self.right_child = None # right child node
class Decision_tree:
"""
Decision tree with binary features
"""
def __init__(self,min_entropy):
self.min_entropy = min_entropy # min node entropy
self.root = None
def fit(self,train_x,train_y):
# construct the decision-tree with recursion
self.root = self.generate_tree(train_x,train_y)
def predict(self,test_x):
# iterate through all samples
prediction = np.zeros([len(test_x),]).astype('int') # placeholder
for i in range(len(test_x)):
#pass # placeholder
node = self.root
data = test_x[i,:]
while node.left_child or node.right_child:
if data[node.feature] == 1:
node = node.right_child
else:
node = node.left_child
prediction[i] = node.label
return prediction
def generate_tree(self,data,label):
# initialize the current tree node
cur_node = Tree_node()
# compute the node entropy
node_entropy = self.compute_node_entropy(label)
if node_entropy < self.min_entropy:
cur_node.isleaf = True
mostFrequent = np.argmax(np.bincount(label))
cur_node.label = mostFrequent
return cur_node
# select the feature that will best split the current non-leaf node
selected_feature = self.select_feature(data,label)
cur_node.feature = selected_feature
# split the data based on the selected feature and start the next level of recursion
featureData = data[:,selected_feature]
zeros = np.asarray(np.where(featureData == 0))[0]
ones = np.asarray(np.where(featureData == 1))[0]
leftData = data[zeros]
leftLabel = label[zeros]
rightData = data[ones]
rightLabel = label[ones]
cur_node.left_child = self.generate_tree(leftData, leftLabel)
cur_node.right_child = self.generate_tree(rightData, rightLabel)
return cur_node
def select_feature(self,data,label):
# iterate through all features and compute their corresponding entropy
best_feat = 0
lowestEntropy = float("inf")
for i in range(len(data[0])):
featureData = data[:,i]
zeros = np.asarray(np.where(featureData == 0))
ones = np.asarray(np.where(featureData == 1))
zerosLabels = label[zeros[0]]
onesLabels = label[ones[0]]
entropy = self.compute_split_entropy(zerosLabels, onesLabels)
if entropy < lowestEntropy:
lowestEntropy = entropy
best_feat = i
return best_feat
def compute_split_entropy(self,left_y, right_y):
# compute the entropy of a potential split, left_y and right_y are labels for the two branches
split_entropy = 0 # placeholder
leftEntropy = self.compute_node_entropy(left_y)
rightEntropy = self.compute_node_entropy(right_y)
leftSamples = len(left_y)
rightSamples = len(right_y)
total = leftSamples + rightSamples
split_entropy = leftEntropy * leftSamples/total + rightEntropy * rightSamples/total
return split_entropy
def compute_node_entropy(self,label):
# compute the entropy of a tree node (add 1e-15 inside the log2 when computing the entropy to prevent numerical issue)
node_entropy = 0 # placeholder
e = 1e-12
totalSamples = len(label)
count = np.bincount(label)
count = count / totalSamples
for j in range(len(count)):
node_entropy += -count[j] * np.log2(count[j] + e)
return node_entropy
Similar Samples
At ProgrammingHomeworkHelp.com, explore our curated samples of programming assignments spanning Java, Python, C++, and more. These examples demonstrate our capability to deliver precise and efficient solutions tailored to academic requirements. Discover how our expertise can assist you in overcoming programming challenges and achieving your academic goals.
Python
Python
Python
Python
Python
Python
Python
Python
Python
Python
Python
Python
Python
Python
Python
Python
Python
Python
Python
Python