Instructions
Objective
Write a python assignment to implement the neighbor-joining algorithm in Python. This algorithm constructs phylogenetic trees, crucial in evolutionary biology. Develop a program that takes a distance matrix, identifies closest neighbors iteratively, and builds the tree. This assignment hones Python skills while solving a practical biological problem.
Requirements and Specifications
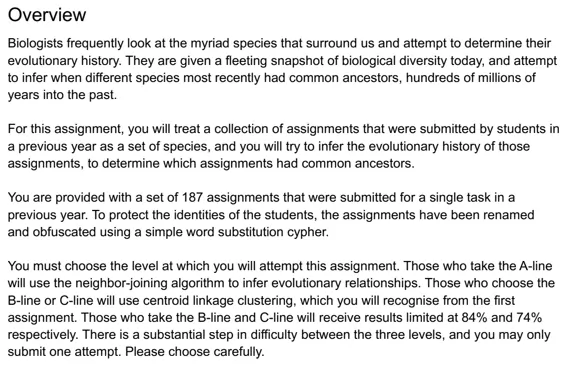
Source Code
# -*- coding: utf-8 -*-
"""
@author:
"""
# do not change or add any import statements
from os.path import basename
from glob import glob
import numpy as np
import matplotlib.pyplot as plt
from scipy.cluster.hierarchy import dendrogram
# %%
def read(filename, n=3):
"""
Please fill in this docstring template
Parameters
----------
filename : str
String containing the file name
n : int, optional
number of the n-grams. The default is 3.
Returns
-------
ngrams : list of tuples
List of tuples where each tuple contain n words
"""
# Open file
ngrams = list()
with open(filename, 'r') as file:
# Read content
content = file.read()
# Split into words
words = content.split(" ")
# Now, construct the ngrams
for i in range(len(words)-n+1):
sublst = list()
for j in range(i, i+n):
w = words[j].strip()
sublst.append(w)
ngrams.append(tuple(sublst))
return ngrams
# %%
def vocabulary(ngrams_list):
"""
Please fill in this docstring template
Parameters
----------
ngrams_list : list of tuples
list of tuples containing the ngrams obtained from the function read(filename, n=3)
Returns
-------
ret: list of tuples
Returns a list of the unique tuples in ngrams
"""
ret = set()
for ngram in ngrams_list:
for tup in ngram:
ret.add(tup)
return list(ret)
# %%
def vectorize(ngrams, vocabulary):
"""
Please fill in this docstring template
Parameters
----------
ngrams : list of tuples
list of n-grams (obtained from 'read')
vocabulary : list of tuples
list of n-grams obtained from 'vocabulary'
Returns
-------
features : NumPy array
NumPy array with the same length of vocabulary, containing 1 and zeros
"""
# Get the length of vocabulary
n = len(vocabulary)
# Create the array filled with zeros
features = np.zeros(n)
# Iterate through the tuples in vocabulary
i = 0
for tup in vocabulary:
if tup in ngrams:
features[i] = 1
i += 1
return features
# %%
def tfidf(vectors):
"""
Please fill in this docstring template
Parameters
----------
vectors : Numpy Array
Numpy Array with 1 and 0
Returns
-------
result : TYPE
DESCRIPTION.
"""
N = len(vectors)
n = len(vectors[0])
tfidf_ret = np.zeros((N, n))
result = np.zeros((N, n))
for i in range(len(vectors)):
vector = vectors[i]
for j in range(len(vector)):
tf = vector[j]
nt = list(vector[:j+1]).count(tf)
idf = np.log(N/(1 + nt))+1
tfidf = tf*idf
tfidf_ret[i,j] = tfidf
return tfidf_ret
# %%
def dissimilarity(v1, v2):
"""
Please fill in this docstring template
Parameters
----------
v1 : TYPE
DESCRIPTION.
v2 : TYPE
DESCRIPTION.
Returns
-------
TYPE
DESCRIPTION.
"""
sumv1_2 = np.sum(np.power(v1, 2))
sumv2_2 = np.sum(np.power(v2,2))
sum = 0
for i in range(len(v1)):
sum += v1[i]*v2[i]
d = 1 - sum/(sumv1_2*sumv2_2)**(1/2)
return d
# %%
def distance_matrix(vectors):
"""
Please fill in this docstring template
Parameters
----------
vectors : TYPE
DESCRIPTION.
Returns
-------
distances : TYPE
DESCRIPTION.
"""
n = len(vectors)
d = np.zeros((n,n))
for i in range(n):
for j in range(n):
v1 = vectors[i]
v2 = vectors[j]
d[i,j] = dissimilarity(v1,v2)
return d
# %%
def nearest_pair(distances, mask):
"""
Please fill in this docstring template
Parameters
----------
distances : 2-D Numpy Array
Numpy Array containing the dissimilarity values
mask : List of boolean
List of boolean values
Returns
-------
pair : List
List with two values containing the index of the smallest values in distances such that maks[i] = mask[j] = False
"""
n = distances.shape[0]
smallest = np.inf
ret = []
for i in range(n):
for j in range(n):
if i != j and distances[i,j] < smallest and mask[i] == False and mask[j] == False:
smallest = distances[i,j]
ret = [i,j]
return ret
# %%
def merge_pair(vectors, linkage, mask, pair):
"""
Please fill in this docstring template
Parameters
----------
vectors : TYPE
DESCRIPTION.
linkage : TYPE
DESCRIPTION.
mask : TYPE
DESCRIPTION.
pair : TYPE
DESCRIPTION.
Returns
-------
None.
"""
# First, select the vectors defined in the pair of index
v1 = vectors[pair[0]]
v2 = vectors[pair[1]]
# Merge vectors
v3 = (v1+v2)/2
np.append(vectors, v3)
# Calculate the distance between the merged vectors, and the average between each merged vector to the new one
dv1v2 = dissimilarity(v1,v2)/2.0
davg = (dissimilarity(v1, v3) + dissimilarity(v2, v3))/2.0
dmax = dv1v2
if davg > dmax:
dmax = davg
# Create new linkage row
linkage_row = [pair[0], pair[1], dmax, len(pair)]
linkage.append(linkage_row)
mask[pair[0]] = True
mask[pair[1]] = True
mask.append(False)
# %%
def update_distance_matrix(distances, vectors):
"""
Please fill in this docstring template
Parameters
----------
distances : TYPE
DESCRIPTION.
vectors : TYPE
DESCRIPTION.
Returns
-------
new_distances : TYPE
DESCRIPTION.
"""
# Pick the last vector
v = vectors[-1]
# Create a new distance matrix, with one more row and column
new_dist = np.zeros((len(vectors), len(vectors)))
# Copy the first N-1 elements into the new matrix
# Fill the elements
for i in range(len(vectors)-1):
for j in range(len(vectors)-1):
new_dist[i,j] = distances[i,j]
# Now, compute the distances to the new vector
for i in range(len(vectors)):
v_ = vectors[i]
new_dist[len(vectors)-1, i] = dissimilarity(v, v_)
new_dist[i, len(vectors)-1] = dissimilarity(v, v_)
return new_dist
# %%
def cluster(vectors):
"""
Please fill in this docstring template
Parameters
----------
vectors : TYPE
DESCRIPTION.
Returns
-------
TYPE
DESCRIPTION.
"""
# First, compute the distance matrix
distances = distance_matrix(vectors)
linkage = []
mask = [False for i in range(len(vectors))]
# Repeat until no pair of vectors needs to be merged
while True:
pair = nearest_pair(distances, mask)
if not pair:
break
merge_pair(vectors, linkage, mask, pair)
new_distances = update_distance_matrix(distances, vectors)
distances = new_distances.copy()
return linkage
# %%
# Do not delete or change code beneath this line.
def main():
"""
Run a clustering analysis on the assignments in the "assignments"
directory, which should be in your current working directory.
Displays the resulting heirarchy as a dendrogram.
Raises
------
RuntimeError
Raised if it can't find the assignments.
Returns
-------
fig : a matplotlib figure
A dendrogram of the heirarchy.
"""
# read in the assignments
data = []
labels = []
files = list(glob('assignments/*'))
if not files:
raise RuntimeError("I can't find the assignments. "
"Are they in your current working directory?")
# Uncomment the following line to test on a subset of assignments
# files = np.random.choice(glob('assignments/*'), size=50, replace=False)
for filename in files:
print(f"Reading and analyzing {filename}... ")
data.append(read(filename))
labels.append(basename(filename)[:-4])
# extract the vocab and convert assignments to vectors
print("Calculating vocabulary...")
vocab = vocabulary(data)
print("Vectorizing...")
vectors = [vectorize(d, vocab) for d in data]
# transform the features to make rare features more important
print("Calculating tfidf...")
vectors = tfidf(vectors)
# cluster the assignments
print("Calculating linkage...")
linkage = cluster(vectors)
# plot the result
print("Plotting...")
fig = plt.figure(figsize=(10, 10))
dendrogram(linkage, labels=labels, orientation='left')
return fig
main()
Related Samples
Read our free Python assignment samples to gain clarity on complex topics. These detailed examples provide valuable insights and solutions, enhancing your understanding and boosting your academic performance.
Python
Python
Python
Python
Python
Python
Python
Python
Python
Python
Python
Python
Python
Python
Python
Python
Python
Python
Python
Python