Instructions
Requirements and Specifications
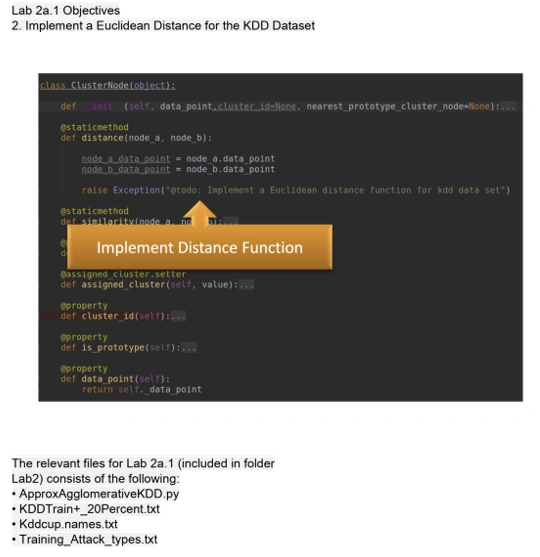
Source Code
import operator
import os
import pandas as pd
import time
import numpy as np
import matplotlib.pyplot as plt
import matplotlib.cm as cm
from collections import defaultdict
from sklearn.preprocessing import StandardScaler
from sklearn.decomposition import PCA
MAX_DISTANCE = 5000
MAX_DISTANCE_FROM_PROTOTYPE = 15
MAX_CLUSTER_DISTANCE = 25
header_names = ['duration', 'protocol_type', 'service', 'flag', 'src_bytes', 'dst_bytes', 'land', 'wrong_fragment', 'urgent', 'hot', 'num_failed_logins', 'logged_in', 'num_compromised', 'root_shell', 'su_attempted', 'num_root', 'num_file_creations', 'num_shells', 'num_access_files', 'num_outbound_cmds', 'is_host_login', 'is_guest_login', 'count', 'srv_count', 'serror_rate', 'srv_serror_rate', 'rerror_rate', 'srv_rerror_rate', 'same_srv_rate', 'diff_srv_rate', 'srv_diff_host_rate', 'dst_host_count', 'dst_host_srv_count', 'dst_host_same_srv_rate', 'dst_host_diff_srv_rate', 'dst_host_same_src_port_rate', 'dst_host_srv_diff_host_rate', 'dst_host_serror_rate', 'dst_host_srv_serror_rate', 'dst_host_rerror_rate', 'dst_host_srv_rerror_rate', 'attack_type', 'success_pred']
kdd_training_file = os.path.join("NSL-KDD", 'KDDTrain+_20Percent.txt')
category = defaultdict(list)
category['benign'].append('normal')
with open('NSL-KDD/training_attack_types.txt', 'r') as f:
for line in f.readlines():
attack, cat = line.strip().split(' ')
category[cat].append(attack)
attack_mapping = dict((v,k) for k in category for v in category[k])
print(repr(attack_mapping))
class ClusterNode(object):
def __init__(self, data_point,cluster_id=None, nearest_prototype_cluster_node=None):
# data point
self._data_point = data_point
self._assigned_cluster = None
# prototype node this node is associated with;
self._nearest_prototype_cluster_node = nearest_prototype_cluster_node
@staticmethod
def distance(node_a, node_b):
node_a_data_point = node_a.data_point
node_b_data_point = node_b.data_point
distance = np.linalg.norm(node_a_data_point - node_b_data_point)
# raise Exception("@todo: Implement a Euclidean distance function for kdd data set")
return distance
@staticmethod
def similarity(node_a, node_b):
return 1 / (1 + ClusterNode.distance(node_a, node_b))
@property
def assigned_cluster(self):
"""
Cluster that this node belongs to
:return:
"""
return self._assigned_cluster
@assigned_cluster.setter
def assigned_cluster(self, value):
self._assigned_cluster = value
@property
def cluster_id(self):
if self._assigned_cluster is None:
return None
return self._assigned_cluster.cluster_id
def print_stuff(self):
print(self.data_point)
@property
def is_prototype(self):
return self == self._nearest_prototype_cluster_node
@property
def data_point(self):
return self._data_point
@property
def nearest_prototype_cluster_node(self):
return self._nearest_prototype_cluster_node
@nearest_prototype_cluster_node.setter
def nearest_prototype_cluster_node(self, value):
self._nearest_prototype_cluster_node = value
class Cluster(object):
def __init__(self, cluster_node_list=[]):
self._cluster_id = id(self)
self._cluster_node_list = []
for cluster_node in cluster_node_list:
self.add_node_to_cluster(cluster_node)
def add_node_to_cluster(self, cluster_node):
# U pdate node's cluster assignment
cluster_node.assigned_cluster = self
# Add node to cluster's node list
self._cluster_node_list.append(cluster_node)
def merge_cluster(self, cluster_2_merge):
for cluster_2_merge_node in cluster_2_merge.cluster_nodes:
self.add_node_to_cluster(cluster_2_merge_node)
@property
def cluster_nodes(self):
for cluster_node in self._cluster_node_list:
yield cluster_node
@staticmethod
def complete_linkage_distance(cluster_a, cluster_b):
"""
Computes the complete linkage distance of two clusters
:param cluster_a:
:param cluster_b:
:return:
"""
# Note: Complete linkage computes the maximum distance of a pair of nodes from cluster a
# and cluster b
max_distance = 0
for cluster_a_node in cluster_a.cluster_nodes:
for cluster_b_node in cluster_b.cluster_nodes:
distance = ClusterNode.distance(cluster_a_node, cluster_b_node)
# If the distance between the current pair of notes is greater than max distance,
# the current distance is the new max distance
if distance > max_distance:
max_distance = distance
return max_distance
@property
def cluster_id(self):
return self._cluster_id
class ApproxAgglomerativeClusteringKDD(object):
def __init__(self, kdd_training_file_path):
self._data_set = None
self._kdd_training_file_path = kdd_training_file_path
self._cluster_node_list = []
self._cluster_list = []
self._is_data_set_loaded = False
self._train_x_continuous_std = None
self._train_Y = None
self._train_x_pca = None
self._pca = None
def plot_training_data(self):
# Check if data set already loaded
if not self._is_data_set_loaded:
self.load_dataset_from_file()
plt.figure()
colors = cm.rainbow(np.linspace(0, 1, len(category)))
for color, cat in zip(colors, category.keys()):
plt.scatter(self._train_x_pca[self._train_Y == cat, 0], self._train_x_pca[self._train_Y == cat, 1],
color=color, alpha=.8, lw=2, label=cat)
plt.legend(loc='right', shadow=False, scatterpoints=1)
plt.show()
def load_dataset_from_file(self):
if self._is_data_set_loaded:
return
train_df = pd.read_csv(self._kdd_training_file_path, names=header_names)
# Aggregates the attack types into categories
train_df['attack_category'] = train_df['attack_type'].map(lambda x: attack_mapping[x])
self.se = train_df['attack_category']
# Remove the attack type labels
train_x_raw = train_df.drop(['attack_type', 'attack_category'], axis=1)
# Remove the features from set that are not continuous
# raise Exception("Need to create a training data set with only continuous features")
symbolic=['attack_category','attack_type','protocol_type','service','flag','land','logged_in','root_shell','su_attempted','is_host_login','is_guest_login']
train_x_continuous = train_df.drop(symbolic,axis=1) # Training data set with only continuous features
# Standardize the data
standard_scaler = StandardScaler().fit(train_x_continuous)
self._train_x_continuous_std = standard_scaler.transform(train_x_continuous)
self._pca = PCA(n_components=2)
self._train_x_pca = self._pca.fit_transform(self._train_x_continuous_std)
self._cluster_node_list = [ClusterNode(data_point) for data_point in self._train_x_continuous_std]
self._is_data_set_loaded = True
def perform_clustering(self):
# Step 1: Determine the prototypes
prototype_list = self._get_prototypes()
# Step 2: Cluster Prototypes
self._cluster_list = self.cluster_nodes(prototype_list)
counter = 0
number_nodes = len(self._cluster_node_list)
# Step 3: Propagate Cluster assignments to nearest prototype
for cluster_node in self._cluster_node_list:
counter +=1
if cluster_node.is_prototype:
continue
# Get cluster assignment from nearest prototype
nearest_prototype_assigned_cluster = cluster_node.nearest_prototype_cluster_node.assigned_cluster
# Add cluster node to cluster
nearest_prototype_assigned_cluster.add_node_to_cluster(cluster_node)
if counter%10 ==0:
print("\n[ {}/{} ] Cluster node {} assigned to cluster {}".format(counter,number_nodes,
repr(cluster_node.data_point),
nearest_prototype_assigned_cluster.cluster_id))
def cluster_nodes(self, cluster_node_list):
# Note: Initially, every cluster node is its own cluster in agglomerative hierarchical clustering
cluster_list = [Cluster([cluster_node]) for cluster_node in cluster_node_list]
while True:
print("\n**** Current number of clusters: {}*****".format(len(cluster_list)))
# Step 1: Compute distances of the clusters
cluster_distance_dict = self._compute_cluster_distances(cluster_list)
# Step 2: Get the minimum cluster distances
(min_cluster_pair, min_cluster_distance_value) = self.get_min_cluster_distance(cluster_distance_dict)
print(
"Minimum Distance of {} is between current cluster {} and cluster {}".format(min_cluster_distance_value,
min_cluster_pair[
0].cluster_id,
min_cluster_pair[
1].cluster_id))
if min_cluster_distance_value >= MAX_CLUSTER_DISTANCE:
break
# Step 3: Merge the min pair clusters
min_cluster_a = min_cluster_pair[0]
min_cluster_b = min_cluster_pair[1]
min_cluster_a.merge_cluster(min_cluster_b)
print("Merging cluster {} into cluster {}; Distance: {}".format(min_cluster_b.cluster_id,
min_cluster_a.cluster_id,
min_cluster_distance_value))
cluster_list.remove(min_cluster_b)
return cluster_list
def _compute_cluster_distances(self, cluster_list):
cluster_distance_dict = dict()
for cluster_a in cluster_list:
cluster_distance_dict[cluster_a] = {}
for cluster_b in cluster_list:
distance = Cluster.complete_linkage_distance(cluster_a, cluster_b)
# print("Distance between current cluster {} and cluster {} is {}".format(cluster_a.cluster_id,
# cluster_b.cluster_id,
# distance))
cluster_distance_dict[cluster_a][cluster_b] = distance
return cluster_distance_dict
def plot_clusters(self):
num_clusters = len(self._cluster_list)
# Plotting
plt.figure()
colors = cm.rainbow(np.linspace(0, 1, num_clusters))
cluster_index = 0
for color, cluster in zip(colors, self._cluster_list):
X = []
Y = []
# for shape in self._train_x_pca:
# print(repr(shape))
for cluster_node in cluster.cluster_nodes:
# x = self._train_x_pca[cluster_node.data_point_index][0]
# y = self._train_x_pca[cluster_node.data_point_index][1]
transformed_data_point = self._pca.transform(cluster_node.data_point.reshape(1,-1))
X.append(transformed_data_point.item(0))
Y.append(transformed_data_point.item(1))
plt.scatter(X, Y, s=50, c=color, marker='o', label="cluster {}".format(cluster_index + 1))
plt.legend(loc='right', shadow=False, scatterpoints=1)
cluster_index += 1
plt.legend(scatterpoints=1)
plt.grid()
plt.show()
pass
def _get_prototypes(self):
prototype_set = set()
prototype_distance_map = dict()
# Initalize prototype distance map, where the key is the prototype and the
# value is that prototypes distance.
# Note: The distance will be initialized to MAX_DISTANCE, which is the maximum distance
prototype_distance_map = {key: value for (key, value) in
[(cluster_node, MAX_DISTANCE) for cluster_node in self._cluster_node_list]}
pass
(curr_max_distance_cluster_node, current_max_distance_value) = self.compute_max_distance_from_prototype(
prototype_distance_map)
while True:
print("\nCurrent max distance: {}".format(current_max_distance_value))
# All of the elements are less than 'MAX_DISTANCE_FROM_PROTOTYPE' away from a prototype
if current_max_distance_value < MAX_DISTANCE_FROM_PROTOTYPE:
break
for cluster_node in self._cluster_node_list:
if cluster_node == curr_max_distance_cluster_node or cluster_node in prototype_set:
continue
# if the current distance from a prototype is greater than that of the curr_max_distance_analysis_report,
# then we'll use the curr_max_distance_analysis_reports distance
dist_from_curr_max_dist_cluster_node = ClusterNode.distance(curr_max_distance_cluster_node,
cluster_node)
if prototype_distance_map[cluster_node] > dist_from_curr_max_dist_cluster_node:
prototype_distance_map[cluster_node] = dist_from_curr_max_dist_cluster_node
# update the clusters nodes nearest prototype
cluster_node.nearest_prototype_cluster_node = curr_max_distance_cluster_node
# The current prototype candidate is its own prototype
curr_max_distance_cluster_node.nearest_prototype_cluster_node = curr_max_distance_cluster_node
# Add the current_prototype candidate to the prototype list
prototype_set.add(curr_max_distance_cluster_node)
print("(Current total:{}) Added cluster node as prototype".format(len(prototype_set)))
# Since the candidate is a prototype, its distance to a prototype is 0 because it is a prototype
prototype_distance_map[curr_max_distance_cluster_node] = 0
# Find the next potential prototype candidate
(curr_max_distance_cluster_node, current_max_distance_value) = self.compute_max_distance_from_prototype(
prototype_distance_map)
return prototype_set
@staticmethod
def compute_max_distance_from_prototype(prototype_distance_map):
""" Returns (key, value) tuple where the value is the max value in dictionary
:param prototype_distance_map:
:return: (key, value) tuple where the value is the max value in dictionary
"""
max_distance = max(prototype_distance_map.items(), key=operator.itemgetter(1))
return max_distance
@staticmethod
def get_min_cluster_distance(cluster_distance_dict):
min_cluster_distance = None
min_cluster_pair = ()
for cluster_key_a in cluster_distance_dict:
for cluster_key_b in cluster_distance_dict[cluster_key_a]:
if cluster_key_a == cluster_key_b:
continue
cluster_distance = cluster_distance_dict[cluster_key_a][cluster_key_b]
if min_cluster_distance is None or min_cluster_distance > cluster_distance:
min_cluster_distance = cluster_distance
min_cluster_pair = (cluster_key_a, cluster_key_b)
return (min_cluster_pair, min_cluster_distance)
@property
def cluster_list(self):
return self._cluster_list
def test_harness():
approx_clustering_kdd = ApproxAgglomerativeClusteringKDD(kdd_training_file)
# Load the dataset
approx_clustering_kdd.load_dataset_from_file()
approx_clustering_kdd.plot_training_data()
start_time = time.time()
approx_clustering_kdd.perform_clustering()
elapsed_time = time.time() - start_time
approx_clustering_kdd.plot_clusters()
print("\nRuntime: {} seconds".format(elapsed_time))
#approx_clustering.plot()
pass
if __name__ == "__main__":
test_harness()
Related Samples
Check out our Python Assignments Sample Section for expertly crafted solutions. From introductory exercises to advanced projects, explore annotated code snippets covering essential concepts like data structures, algorithms, and more. Perfect for students seeking clarity and proficiency in Python programming.
Python
Python
Python
Python
Python
Python
Python
Python
Python
Python
Python
Python
Python
Python
Python
Python
Python
Python
Python
Python