Instructions
Objective
Write a python assignment program to implement digit classification.
Requirements and Specifications
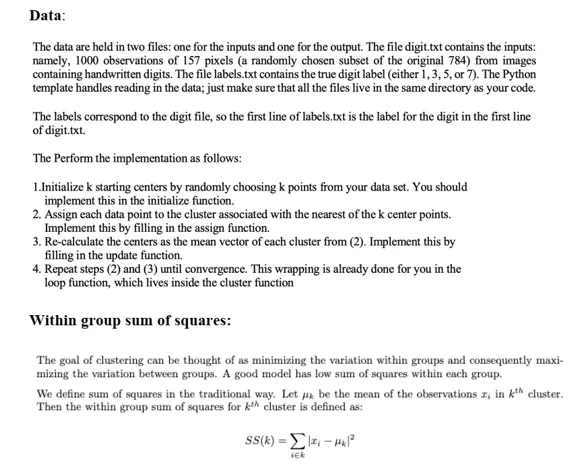
Source Code
"""
K-means clustering.
"""
import numpy as np
import pandas as pd
from matplotlib import pyplot as plt
def analyze_kmeans():
"""
Top-level wrapper to iterate over a bunch of values of k and plot the
distortions and misclassification rates.
"""
X = np.genfromtxt("digit.txt")
y = np.genfromtxt("labels.txt", dtype=int)
distortions = []
errs = []
ks = range(1, 11)
for k in ks:
distortion, err = analyze_one_k(X, y, k)
distortions.append(distortion)
errs.append(err)
fig, ax = plt.subplots(2, figsize=(8, 6))
ax[0].plot(ks, distortions, marker=".")
ax[0].set_ylabel("Distortion")
ax[1].plot(ks, errs, marker=".")
ax[1].set_xlabel("k")
ax[1].set_ylabel("Mistake rate")
ax[0].set_title("k-means performance")
fig.savefig("kmeans.png")
def analyze_one_k(X, y, k):
"""
Run the k-means analysis for a single value of k. Return the distortion and
the mistake rate.
"""
print("Running k-means with k={0}".format(k))
clust = cluster(X, y, k)
print("Computing classification error.")
err = compute_mistake_rate(y, clust)
return clust["distortion"][0], err
def cluster(X, y, k, n_starts=5):
"""
Run k-means a total of n_starts times. Returns the results from the run that
had the lowest within-group sum of squares (i.e. the lowest distortion).
Inputs
------
X is an NxD1 matrix of inputs.(D1=157)
y is a D2x1 vector of labels.(D2=1000)
n_starts says how many times to randomly re-initialize k-means. You don't
need to change this.
Outputs
-------
The output is a dictionary with the following fields:
Mu is a kxD1 matrix of cluster centroids
z is an Nx1 vector assigning points to clusters. So, for instance, if z[4] =
2, then the algorithm has assigned the 4th data point to the second
cluster.
distortion is the within-group sum of squares, a number.
"""
def loop(X, i):
"""
A single run of clustering.
"""
Mu = initialize(X, k) #Done
N = X.shape[0]
z = np.repeat(-1, N) # So that initially all assignments change.-1 repeats N times
while True:
old_z = z
z = assign(X, Mu) # The vector of assignments z.
Mu = update(X, z, k) # Update the centroids
if np.all(z == old_z):
distortion = compute_distortion(X, Mu, z)
return dict(Mu=Mu, z=z, distortion=distortion)
# Main function body
print("Performing clustering.")
results = [loop(X, i) for i in range(n_starts)]
best = min(results, key=lambda entry: entry["distortion"])
best["digits"] = label_clusters(y, k, best["z"])
return best
def assign(X, Mu):
"""
Assign each entry to the closest centroid. Return an Nx1 vector of
assignments z.
X is the NxD matrix of inputs.
Mu is the kxD matrix of cluster centroids.
"""
z=[]
for i in range(0,len(X)):
dist=[]
for j in range(0,len(Mu)):
dist.append(np.linalg.norm(X[i]-Mu[j]))
z.append(dist.index(min(dist)))
return z
def update(X, z, k):
"""
Update the cluster centroids given the new assignments. Return a kxD matrix
of cluster centroids Mu.
X is the NxD inputs as always.
z is the Nx1 vector of cluster assignments.
k is the number of clusters.
"""
# TODO: Compute the cluster centroids Mu.
b=X.shape[1]
Mu=np.zeros(shape=(k,b))
for i in range(0,k):
cluster_index=[]
for j in range(0,(len(z))):
if z[j]==i:
cluster_index.append(j)
Mu[i,:]=np.mean(X[cluster_index,:],axis=0)
return Mu
def compute_distortion(X, Mu, z):
"""
Compute the distortion (i.e. within-group sum of squares) implied by NxD
data X, kxD centroids Mu, and Nx1 assignments z.
"""
# TODO: Compute the within-group sum of squares (the distortion).
k=Mu.shape[0]
distortion = []
for i in range(0,k):
cluster_index=[]
for j in range(0,(len(z))):
if z[j]==i:
cluster_index.append(j)
distortion1=0
for j in cluster_index:
distortion1= distortion1 + (np.linalg.norm(X[j]-Mu[i])**2)
distortion.append(distortion1)
return distortion
def initialize(X, k):
"""
Randomly initialize the kxD matrix of cluster centroids Mu. Do this by
choosing k data points randomly from the data set X.
"""
index = np.random.choice(len(X),k)
Mu=X[index,:]
return Mu
def label_clusters(y, k, z):
"""
Label each cluster with the digit that occurs most frequently for points
assigned to that cluster.
Return a kx1 vector labels with the label for each cluster.
For instance: if 20 points assigned to cluster 0 have label "3", and 40 have
label "5", then labels[0] should be 5.
y is the Nx1 vector of digit labels for the data X
k is the number of clusters
z is the Nx1 vector of cluster assignments.
"""
# TODO: Compute the cluster labelings.
labels=[]
for i in range(0,k):
print("i",i)
list_1=[]
cluster_index=[]
for j in range(0,(len(z))):
if z[j]==i:
cluster_index.append(j)
list_1 = y[cluster_index]
label_data=pd.DataFrame(data=list_1,columns=["Y"])
labels.append(label_data['Y'].value_counts().idxmax())
return np.array(labels)
def compute_mistake_rate(y, clust):
"""
Compute the mistake rate as discussed in section 3.4 of the homework.
y is the Nx1 vector of true labels.
clust is the output of a run of clustering. Two fields are relevant:
"digits" is a kx1 vector giving the majority label for each cluster
"z" is an Nx1 vector of final cluster assignments.
"""
print("start compute")
clust['z']=np.array(clust['z'])
def zero_one_loss(xs, ys):
return sum(xs != ys) / float(len(xs))
y_hat = clust["digits"][clust["z"]]
return zero_one_loss(y, y_hat)
def main():
analyze_kmeans()
if __name__ == '__main__':
main()
Similar Samples
At ProgrammingHomeworkHelp.com, we provide a diverse range of sample programming assignments that highlight our expertise. Explore our samples to see how we excel in delivering accurate and well-structured solutions across various programming languages. Whether you need help with Java, Python, or any other language, our samples demonstrate our commitment to quality and proficiency in programming homework assistance.
Python
Python
Python
Python
Python
Python
Python
Python
Python
Python
Python
Python
Python
Python
Python
Python
Python
Python
Python
Python