Instructions
Objective
Write a program to implement convolution network in python.
Requirements and Specifications
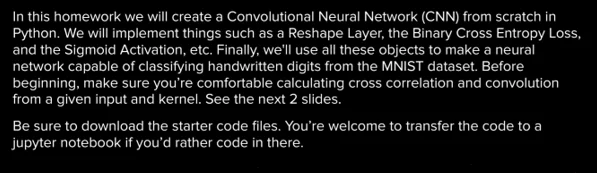
Source Code
ACTIVISION
import numpy as np
from layer import Layer
class Activation(Layer):
def __init__(self, activation, activation_prime):
self.activation = activation
self.activation_prime = activation_prime
def forward(self, input):
self.input = input
return self.activation(self.input)
def backward(self, output_gradient, learning_rate):
return np.multiply(output_gradient, self.activation_prime(self.input))
CONVOLUTION
import numpy as np
from scipy import signal
from layer import Layer
class Convolutional(Layer):
def __init__(self, input_shape, kernel_size, depth):
input_depth, input_height, input_width = input_shape
self.depth = depth
self.input_shape = input_shape
self.input_depth = input_depth
self.output_shape = (depth, input_height - kernel_size + 1, input_width - kernel_size + 1)
self.kernels_shape = (depth, input_depth, kernel_size, kernel_size)
self.kernels = np.random.randn(*self.kernels_shape)
self.biases = np.random.randn(*self.output_shape)
def forward(self, input):
self.input = input
self.output = np.copy(self.biases)
# TODO: Implement the forward method using the formula provided in the powerpoint.
# You may add or remove any variables that you wish.
for i in range(self.depth):
for j in range(self.input_depth):
self.output[i] += signal.correlate2d(self.input[j], self.kernels[i, j], "valid")
return self.output
def backward(self, output_gradient, learning_rate):
# TODO: initialize the kernels_gradient and input_gradient.
kernels_gradient = np.zeros(self.kernels_shape)
input_gradient = np.zeros(self.input_shape)
# TODO: implement the back pass here. The equations in the ppt may help, but you're free to
# add as much or as little code as you'd like.
for i in range(self.depth):
for j in range(self.input_depth):
kernels_gradient[i, j] = signal.correlate2d(self.input[j], output_gradient[i], "valid")
input_gradient[j] += signal.convolve2d(output_gradient[i], self.kernels[i, j], "full")
# TODO: update the kernels and biases
self.kernels -= learning_rate * kernels_gradient
self.biases -= learning_rate * output_gradient
return input_gradient
DENSE
import numpy as np
from layer import Layer
class Dense(Layer):
def __init__(self, input_size, output_size):
self.weights = np.random.randn(output_size, input_size)
self.bias = np.random.randn(output_size, 1)
def forward(self, input):
# TODO: apply linear transformation to the input. see ppt for equation.
self.input = input
self.output = (np.dot(self.weights,input) + self.bias)
return self.output
def backward(self, output_gradient, learning_rate):
# TODO: update the weights and bias
weights_gradient = np.dot(output_gradient,self.input.T)
X_gradient = np.dot(self.weights.T,output_gradient)
self.weights -= learning_rate * weights_gradient
self.bias -= learning_rate * output_gradient
return X_gradient
Similar Samples
Explore our expertly crafted programming solutions at ProgrammingHomeworkHelp.com. From Java to Python, our samples showcase top-notch code snippets and comprehensive explanations, perfect for enhancing your programming skills and understanding.
Python
Python
Python
Python
Python
Python
Python
Python
Python
Python
Python
Python
Python
Python
Python
Python
Python
Python
Python
Python