Instructions
Objective
Write a program to create K nearest neighbour classifier in python.
Requirements and Specifications
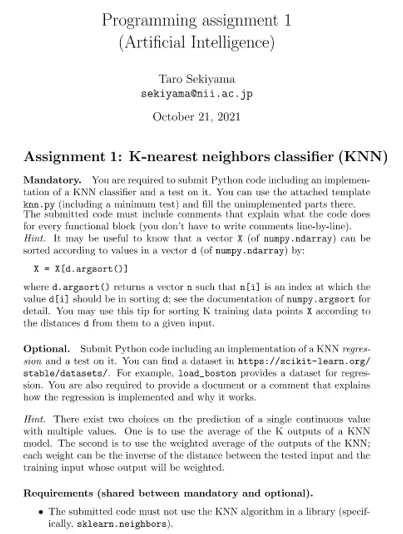
Source Code
# -*- coding: utf-8 -*-
import pandas as pd
import numpy as np
from sklearn.model_selection import train_test_split
import matplotlib.pyplot as plt
data_url = "http://lib.stat.cmu.edu/datasets/boston"
raw_df = pd.read_csv(data_url, sep="\s+", skiprows=22, header=None)
raw_df.head()
data = np.hstack([raw_df.values[::2, :], raw_df.values[1::2, :2]])
target = raw_df.values[1::2, 2]
"""## Split into Train and Test"""
X_train, X_test, y_train, y_test = train_test_split(data, target, test_size = 0.33, random_state = 42)
X_train = np.array([
[5, 45],
[5.11, 26],
[5.6, 30],
[5.9, 34],
[4.8, 40],
[5.8, 36],
[5.3, 19],
[5.8, 28],
[5.5, 23],
[5.6, 32],
])
y_train = np.array([77, 47, 55, 59, 72, 60, 40, 60, 45, 58])
X_test = np.array([
[5.5, 38]
])
y_test = np.array([65.2])
"""## Define function to calculate the euclidean distance"""
def euclidean_distance(X, x):
return np.sqrt(np.sum(np.power(X-x,2), axis=1))
"""## Define K
"""
K = 3
"""## Function to predict"""
def predict(x, X_train, y_train, K):
# Compute euclidean distances
D = euclidean_distance(X_train, x)
# Sort in ascending order
D = np.argsort(D)
# Pick index of last K elements
D_ = D[:K]
# Get target values of the select K elements
y_ = y_train[D_]
# Calculate mean
yi = np.mean(y_)
return yi
"""## Estimate"""
y_pred = np.zeros(y_test.shape)
for i, x in enumerate(X_test):
yi = predict(x, X_train, y_train, K)
# Store predicted value
y_pred[i] = yi
"""## Compute error"""
err = np.sqrt(1/len(y_test) *np.sum(np.power(y_test-y_pred, 2)))
print("The RMSE error is: {:.4f}".format(err))
"""## Compute error for different values of K"""
errors = list()
for k in range(1, 10):
y_pred = np.zeros(y_test.shape)
for i, x in enumerate(X_test):
yi = predict(x, X_train, y_train, k)
y_pred[i] = yi
# Compute error
err = np.sqrt(1/len(y_test) *np.sum(np.power(y_test-y_pred, 2)))
errors.append(err)
# Plot
plt.figure()
plt.plot(range(1, 10), errors)
plt.grid(True)
plt.show()
"""### We see that the optimal value of K is K = 5"""
Related Samples
Explore our collection of free machine learning assignment samples to gain insights into our approach and quality. Each sample showcases our expertise in tackling diverse ML topics effectively. Start exploring today!
Machine Learning
Machine Learning
Machine Learning
Machine Learning
Machine Learning
Machine Learning
Machine Learning
Machine Learning