Claim Your Offer
Unlock an amazing offer at www.programminghomeworkhelp.com with our latest promotion. Get an incredible 10% off on your all programming assignment, ensuring top-quality assistance at an affordable price. Our team of expert programmers is here to help you, making your academic journey smoother and more cost-effective. Don't miss this chance to improve your skills and save on your studies. Take advantage of our offer now and secure exceptional help for your programming assignments.
We Accept
In the ever-evolving landscape of technological advancement, the marriage of machine learning and engineering has emerged as a transformative force, offering unprecedented solutions to the intricate challenges posed by complex engineering problems, including the ability to solve your Machine Learning assignment. At the core of this synergy is the recognition that traditional approaches often fall short in the face of the interconnected variables and vast datasets that define the complexity of engineering dilemmas. Machine learning, with its capacity to analyze massive amounts of data and identify patterns, becomes the linchpin in unraveling the intricacies that often elude conventional problem-solving methodologies. It's a paradigm shift, wherein the algorithms inherent to machine learning sift through the noise, discerning trends, anomalies, and correlations with an acuity that surpasses human intuition.
Predictive analytics, a subset of machine learning, stands out as a beacon in streamlining decision-making processes within engineering domains. By extrapolating insights from historical data, predictive analytics empowers engineers to make informed decisions, whether preemptively averting crises in manufacturing by predicting equipment failures or optimizing supply chain logistics. The foresight provided by machine learning models becomes a cornerstone in the realm of problem-solving, ensuring not only the resolution of immediate issues but also the enhancement of overall operational efficiency.
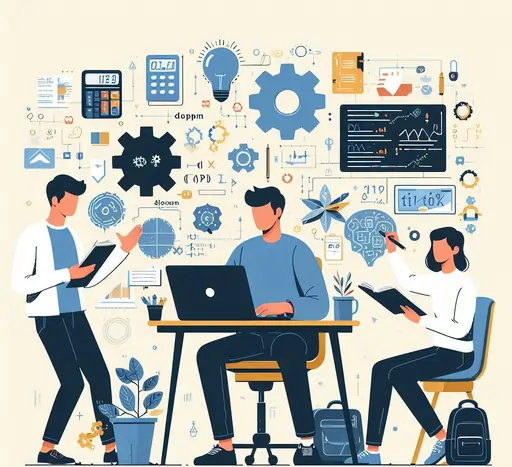
Neural networks, inspired by the complexity of the human brain, bring a level of sophistication to engineering problem-solving that was once deemed implausible. In scenarios demanding pattern recognition and an understanding of nonlinear relationships, neural networks shine. From quality control applications utilizing image recognition to speech processing in autonomous systems, these intricate systems are pushing the boundaries of what was previously achievable, redefining the scope of engineering solutions.
The application of machine learning in simulation and modeling further amplifies its impact on engineering problem-solving. As simulation and modeling play pivotal roles in understanding, testing,
and optimizing designs, the integration of machine learning introduces an element of adaptability and accuracy that was previously unparalleled. Whether simulating fluid dynamics in aerodynamics or optimizing structural designs in civil engineering, machine learning models provide a dynamic approach to problem-solving, learning from data, and offering insights into potential improvements.
In essence, breaking down complex engineering problems with machine learning is an ongoing journey, marked by continuous innovation and discovery. It is a testament to the limitless potential that lies at the intersection of technology and engineering excellence. As we navigate this uncharted territory, the fusion of machine learning and engineering promises not only to meet the challenges posed by complex problems but to redefine the very nature of problem-solving itself. The future holds a landscape where the synergies between machine learning and engineering will likely birth groundbreaking innovations, cementing this integration as an indispensable cornerstone of progress in the realm of technology-driven problem resolution.
The amalgamation of machine learning and engineering stands as a testament to the ever-accelerating pace of technological evolution. This symbiotic relationship is not merely a refinement of existing methodologies, but a paradigm shift that redefines the fundamental approach to addressing complex engineering problems. The intrinsic capability of machine learning to decipher intricate patterns within massive datasets addresses a critical shortfall in traditional problem-solving techniques, offering engineers a dynamic toolkit to navigate the complexities inherent in their fields.
At the heart of this revolution is the recognition that engineering challenges are no longer confined to isolated, linear problems. Instead, they often manifest as intricate webs of interconnected variables, necessitating an approach that transcends the limitations of human cognitive capacity. Machine learning algorithms, fueled by the power of computational analysis, sift through the labyrinthine complexity of data, extracting meaningful insights, and revealing patterns that might otherwise remain obscured.
Predictive analytics, a formidable offspring of machine learning, emerges as a guiding light in the decision-making processes of engineers. By extrapolating trends from historical data, predictive analytics empower decision-makers to anticipate future scenarios, mitigating risks, and optimizing outcomes. This capability is particularly potent in industries where proactive measures are imperative, such as predicting equipment failures in manufacturing or fine-tuning logistical strategies in intricate supply chains. In essence, machine learning becomes the oracle that engineers consult to navigate the uncertainties inherent in their dynamic environments.
Neural networks, mimicking the intricate architecture of the human brain, usher in a new era of problem-solving sophistication. In scenarios demanding the recognition of intricate patterns and the comprehension of nonlinear relationships, neural networks demonstrate unparalleled prowess. From revolutionizing image recognition in quality control processes to enhancing the comprehension of complex speech patterns in autonomous systems, these neural architectures elevate engineering solutions to unprecedented heights. The versatility of neural networks positions them as a cornerstone in addressing challenges that were once considered insurmountable.
The role of machine learning extends beyond pattern recognition; it permeates the realm of simulation and modeling, fundamental aspects of engineering design and optimization. Here, machine learning injects adaptability and precision into the traditionally rigid and deterministic simulation processes. Through learning from vast datasets, machine learning models simulate dynamic systems, offering insights into potential improvements. Whether navigating the fluid dynamics of aerodynamics or
optimizing the structural integrity of civil engineering projects, machine learning transforms simulations from static exercises into dynamic, evolving processes.
As we reflect on the profound implications of breaking down complex engineering problems with machine learning, it becomes evident that this journey is not a destination but a perpetual exploration of possibilities. The synergy between machine learning and engineering is an evolving narrative, marked by continuous innovation and the relentless pursuit of excellence. This integration is not a mere augmentation of existing practices; it is a redefinition of the very essence of engineering problem-solving.
In the grand tapestry of technological progress, the fusion of machine learning and engineering emerges as a crucial thread, weaving together unprecedented solutions and transformative breakthroughs. The future promises a landscape where these synergies will not only meet the challenges posed by complex problems but will reshape the contours of what is conceivable in the realm of technology-driven problem resolution. The journey continues, propelled by the inexhaustible curiosity and ingenuity of those at the forefront of this convergence.
Understanding the Landscape of Complex Engineering Problems
In the intricate world of engineering, navigating the landscape of complex problems is akin to traversing a multifaceted terrain filled with challenges and uncertainties. The inherent complexity of engineering dilemmas arises from the intricate interplay of numerous variables, often entwined in a web of interconnected relationships. From optimizing manufacturing processes to designing efficient supply chain systems, engineers grapple with problems that transcend linear thinking and demand sophisticated solutions. The sheer volume of data generated in engineering endeavors further compounds the intricacy, requiring a level of analytical prowess that traditional methods struggle to achieve.
Machine learning emerges as a beacon of hope in this complex scenario, offering the capability to sift through massive datasets with unparalleled efficiency. It becomes a powerful ally in unraveling the underlying patterns, trends, and correlations that might elude human perception. The nuanced understanding of complex engineering problems facilitated by machine learning is not merely about data processing; it is about gaining insights into the intricacies of dynamic systems, predicting their behavior, and unlocking novel solutions. As industries embrace digital transformation, the symbiotic relationship between machine learning and engineering becomes increasingly apparent, promising a future where the once-daunting landscape of complex problems is navigated with confidence and precision.
In the vast and ever-evolving domain of engineering, where challenges are as diverse as the systems they aim to optimize or create, understanding the landscape of complex problems is a journey through a labyrinth of intricacies. These challenges span a spectrum from fine-tuning intricate manufacturing processes to orchestrating the logistics of a global supply chain with precision. What renders these problems complex is not only their diversity but the intricate web of relationships and dependencies among numerous variables. Engineers face a relentless pursuit of efficiency, innovation, and reliability, and the conventional tools at their disposal often fall short in handling the sheer complexity of these tasks.
Enter machine learning—a revolutionary force that holds the promise of unraveling the complexity inherent in these challenges. The allure of machine learning lies not just in its ability to process colossal volumes of data but in its capacity to discern hidden patterns and relationships within that data. This is a transformative leap beyond the conventional, as it enables engineers to perceive intricate connections, make sense of nonlinear dynamics, and predict outcomes that would otherwise remain obscured.
The marriage of machine learning and engineering is a paradigm shift, elevating problem-solving to new heights by augmenting human capabilities with artificial intelligence. From optimizing energy consumption in smart cities to predicting equipment failures in industrial plants, machine learning becomes the guiding light through the intricate landscape of complex engineering problems. It is not merely a tool but a cognitive companion, enabling engineers to gain profound insights into the inner workings of complex systems. As industries embark on the journey of digital transformation, the demand for solutions to increasingly intricate challenges has never been more pressing. Machine learning emerges not just as a solution but as a transformative force, reshaping the very nature of engineering problem-solving.
The landscape, once daunting and enigmatic, now becomes a canvas for innovation and discovery. The nuanced understanding facilitated by machine learning is not confined to the realm of algorithms and data; it extends to a deep comprehension of the dynamic systems that engineers seek to manipulate and optimize. Through this lens, the landscape of complex engineering problems transforms from an obstacle-laden terrain to a realm of opportunities waiting to be unlocked. The synergy between human expertise and machine learning algorithms creates a potent combination, where the whole is greater than the sum of its parts. The once-daunting landscape, with its intricate problems and convoluted challenges, becomes a canvas for collaborative exploration.
It is a journey where engineers, armed with the power of machine learning, navigate through the complexities with confidence, uncovering solutions that were previously obscured. In this symbiotic relationship, machine learning does not replace human ingenuity; it amplifies it. It acts as a guide, a partner, and a catalyst for innovation. As we stand at the intersection of machine learning and engineering, the landscape of complex problems is no longer an obstacle to overcome but a tapestry to weave together—thread by thread, insight by insight. The journey of understanding this landscape is ongoing, and with each discovery, the boundaries of what is achievable in engineering are pushed further, heralding an era where complex problems are not barriers but gateways to new possibilities.
Streamlining Decision-Making with Predictive Analytics
In the ever-evolving landscape of engineering, the integration of machine learning, particularly through the lens of predictive analytics, has emerged as a pivotal force in revolutionizing decision-making processes. In the face of complex engineering challenges, where the stakes are high and outcomes are often contingent on a multitude of variables, predictive analytics offers a transformative approach. By harnessing the power of historical data, machine learning models can discern patterns and trends that might elude traditional analytical methods. This capability becomes particularly critical when navigating intricate scenarios that demand proactive decision-making. Consider scenarios in manufacturing where the prediction of equipment failures can prevent costly downtime or in supply chain management where anticipating demand fluctuations can optimize inventory levels. Predictive analytics empowers engineers and decision-makers with actionable insights derived from a comprehensive analysis of past data, enabling them to make informed choices that not only mitigate risks but also enhance operational efficiency.
The ability to foresee potential challenges and opportunities through predictive analytics aligns seamlessly with the dynamic and unpredictable nature of engineering endeavors. As industries continue to embrace the digital era, the integration of predictive analytics becomes a cornerstone in reshaping decision-making paradigms, steering the course of engineering practices towards a future defined by foresight, efficiency, and strategic precision.
Predictive analytics, within the realm of machine learning, transcends traditional decision-making paradigms in engineering by offering a dynamic and forward-thinking approach. The essence of this transformative power lies in the model's ability to extrapolate patterns from historical data, thereby allowing engineers to anticipate future scenarios with unprecedented accuracy. In industries where precision and timely responses are paramount, such as aerospace or healthcare, predictive analytics becomes a linchpin in strategic planning and risk mitigation. For instance, in aerospace, predicting the maintenance needs of intricate machinery can prevent catastrophic failures, ensuring the safety of both equipment and personnel. Similarly, in healthcare, predictive analytics can be applied to forecast patient admission rates, enabling hospitals to optimize resource allocation and enhance patient care.
The scalability of predictive analytics extends its reach beyond individual use cases, permeating through diverse engineering domains. Its application in energy management involves forecasting energy consumption patterns, enabling efficient utilization of resources and reducing environmental impact. In urban planning, predictive analytics aids in predicting traffic patterns, facilitating the development of intelligent transportation systems. Moreover, the fusion of predictive analytics with Internet of Things (IoT) devices allows for real-time data analysis, empowering engineers to respond promptly to changing conditions.
The iterative nature of machine learning models ensures continuous improvement, as the system refines its predictions over time with the influx of new data. This adaptive quality makes predictive analytics a dynamic tool for engineers, refining their decision-making processes and allowing for agile responses to evolving circumstances. Furthermore, the interpretability of machine learning models, often considered a challenge, is being addressed through advancements such as explainable AI, providing engineers with insights into the model's decision-making rationale.
The integration of predictive analytics into engineering decision-making not only optimizes operational efficiency but also opens avenues for innovation. Engineers armed with predictive insights are better equipped to explore novel solutions, pushing the boundaries of what was once deemed impossible. The democratization of data-driven decision-making, facilitated by user-friendly interfaces and tools, ensures that the benefits of predictive analytics are accessible across various levels of expertise within engineering teams.
As the symbiotic relationship between machine learning and engineering deepens, predictive analytics stands as a beacon of transformative potential. Its role in enhancing decision-making processes is not merely a technological advancement; it represents a paradigm shift in how engineers approach challenges. In a world where uncertainties abound, predictive analytics emerges as a guiding light, enabling engineers to navigate the complexities of their domains with confidence and foresight. In essence, the marriage of machine learning and engineering decision-making through predictive analytics lays the foundation for a future where precision, efficiency, and innovation converge to shape a new era in engineering excellence.
Enhancing Problem-Solving with Neural Networks
In the realm of engineering, where complexities often defy straightforward solutions, the integration of neural networks stands out as a transformative force in problem-solving. Drawing inspiration from the intricate architecture of the human brain, neural networks bring a level of sophistication that is reshaping traditional approaches to engineering challenges. Their prowess lies in their ability to discern intricate patterns, process vast datasets, and tackle problems that exhibit nonlinear relationships. Unlike conventional programming methods, where explicit rules are defined, neural networks excel in tasks that demand a nuanced understanding of complex data.
From image and speech recognition to predictive modeling, neural networks are demonstrating unprecedented capabilities. In quality control processes, for instance, they can identify defects in manufacturing by recognizing subtle visual patterns that might elude human inspectors. In the realm of autonomous systems, such as self-driving cars, neural networks process and interpret vast amounts of sensory data to make real-time decisions, showcasing their adaptability and learning capacity.
The application of neural networks extends beyond individual problem domains, permeating diverse fields of engineering where their ability to adapt, learn, and generalize from data is harnessed for unparalleled problem-solving. As the demand for more sophisticated and efficient solutions grows, the integration of neural networks into the fabric of engineering problem-solving represents not just a technological leap but a paradigm shift that is defining the future of problem-solving in the complex world of engineering.
Neural networks, with their layered architecture, process information in a manner reminiscent of the human brain, allowing them to recognize and understand intricate patterns that elude conventional algorithms. This adaptability is particularly crucial in scenarios where problems evolve over time or exhibit dynamic characteristics. For instance, in the field of finance, neural networks are employed for predictive modeling of market trends, adjusting their understanding as financial landscapes shift. Moreover, in cybersecurity, neural networks play a pivotal role in detecting and preventing cyber threats by constantly learning from new attack patterns. The applications extend into healthcare, where they aid in diagnosing diseases from medical imaging data, showcasing their potential in revolutionizing diagnostic processes.
As neural networks continue to evolve and scale, their ability to generalize from diverse datasets enables them to address a wide array of engineering challenges, from optimizing energy consumption in smart grids to enhancing the efficiency of robotic systems in industrial automation. The inherent ability of neural networks to learn from experience, adapt to changing conditions, and autonomously improve their performance positions them as a linchpin in the ongoing evolution of problem-solving paradigms within the engineering landscape.
The symbiotic relationship between neural networks and engineering challenges is a testament to the transformative potential that lies at the intersection of artificial intelligence and traditional problem-solving methodologies. As the capabilities of neural networks continue to unfold, their integration into the fabric of engineering problem-solving is not just a technological advancement but a
pivotal step towards unlocking new realms of efficiency, innovation, and understanding in the ever-evolving field of engineering.
The Role of Machine Learning in Simulation and Modeling
The role of machine learning in simulation and modeling within the realm of engineering is a transformative force that has revolutionized the way we understand, test, and optimize complex systems. Traditional simulation methods often struggled to capture the intricate dynamics of real-world scenarios, but machine learning has emerged as a powerful ally, enhancing the precision and efficiency of these processes. Through the integration of sophisticated algorithms and neural networks, machine learning models are adept at learning from data, adapting to changing conditions, and providing valuable insights into potential improvements.
In fields such as aerodynamics, where fluid dynamics simulations are critical, machine learning enables a more nuanced understanding of airflow patterns, contributing to the development of more aerodynamically efficient designs. Similarly, in civil engineering, the optimization of structural designs is made more effective by machine learning models that can analyze vast datasets to identify patterns and correlations, leading to more robust and resilient structures.
The ability of machine learning to simulate and model complex systems extends beyond traditional engineering domains, encompassing diverse areas such as materials science, healthcare, and finance. Whether it's predicting the behavior of new materials, simulating biological processes, or optimizing financial portfolios, machine learning is proving to be a versatile tool that transcends disciplinary boundaries. The fusion of machine learning with simulation and modeling not only accelerates the pace of innovation but also opens up new frontiers in our quest to comprehend and master the complexities of the physical world. As we continue to harness the potential of machine learning in simulation and modeling, the boundaries of what is achievable in engineering and scientific endeavors are continually expanding, ushering in an era of unprecedented insights and advancements.
The integration of machine learning into simulation and modeling processes is fundamentally altering the landscape of engineering by providing a nuanced understanding of intricate systems and fostering a data-driven approach to problem-solving. Unlike traditional simulation methods that rely on explicit programming, machine learning models have the ability to autonomously learn from data, allowing them to adapt and evolve in response to changing conditions. This adaptability is particularly beneficial in scenarios where the underlying dynamics of a system may be complex, nonlinear, or difficult to model using conventional approaches. In fields such as climate science, for instance, where the interactions between various environmental factors are highly intricate, machine learning enables more accurate and dynamic climate models, leading to improved predictions of climate patterns and trends.
In the realm of healthcare, machine learning aids in the simulation and modeling of biological processes, enabling more precise predictions of disease progression and responses to treatments. Drug discovery, for example, benefits from machine learning models that simulate the interactions between various compounds and biological targets, expediting the identification of potential therapeutic agents. The personalized medicine revolution is further propelled by machine learning algorithms that analyze
patient data to simulate the effects of different treatment regimens, guiding clinicians towards more tailored and effective interventions.
Financial institutions leverage machine learning in simulation and modeling for risk assessment, portfolio optimization, and fraud detection. These applications involve the dynamic analysis of vast datasets, identifying patterns and anomalies to inform decision-making. The ability to simulate market conditions, assess the impact of various economic factors, and optimize investment portfolios in real-time positions financial institutions to navigate the complexities of the global economy with greater agility and accuracy.
As machine learning continues to advance, the integration of reinforcement learning, generative models, and other cutting-edge techniques further expands the capabilities of simulations. These advancements open new avenues for exploring complex scenarios, optimizing designs, and gaining insights into previously intractable problems. The collaborative efforts of machine learning researchers and domain experts across various fields are propelling us into an era where the simulation and modeling of complex systems are not only more accurate and efficient but also more accessible and democratized. The ongoing exploration of these frontiers promises to unlock unprecedented potential in engineering, science, and beyond, pushing the boundaries of what is achievable and redefining our approach to solving the most intricate problems.
Conclusion
In the ever-advancing realm of technology, the marriage of machine learning and engineering has emerged as a catalyst for transformative problem-solving. As we navigate the complex landscape of engineering challenges, machine learning stands out as a beacon of innovation, capable of unraveling intricacies that traditional methods find daunting. This integration is particularly pronounced in predictive analytics, where the power of algorithms to sift through vast datasets and discern patterns has revolutionized decision-making processes. The ability to predict outcomes based on historical data not only averts crises but also enhances overall operational efficiency, be it in manufacturing, supply chain logistics, or other dynamic fields.
The advent of neural networks, inspired by the human brain, has ushered in a new era of pattern recognition and nonlinear relationship understanding. From image recognition in quality control to speech processing in autonomous systems, neural networks are pushing the boundaries of engineering problem-solving. Simultaneously, the role of machine learning in simulation and modeling has redefined how engineers understand, test, and optimize designs.
By adapting to changing conditions and learning from data, machine learning models offer unprecedented insights into fluid dynamics, aerodynamics, structural design, and more. As we reflect on the multifaceted impact of machine learning on engineering problem-solving, it becomes clear that we stand at the threshold of a new era. The synergy between technology and engineering excellence is not only reshaping our approach to challenges but also opening doors to groundbreaking innovations. The journey of breaking down complex engineering problems with machine learning is an ongoing saga, and the future promises limitless possibilities for those at the forefront of this intersection of technology and engineering prowess.
This transformative synergy between machine learning and engineering is not merely a technological convergence; it represents a paradigm shift in how we conceptualize and surmount challenges across diverse domains. The intricacies of modern engineering dilemmas, from optimization puzzles to predictive modeling complexities, are met with unparalleled efficacy through the lens of machine learning. The ability of algorithms to traverse vast datasets, identifying subtle patterns and correlations, brings a level of analytical finesse that transcends human capacity. In the dynamic landscape of predictive analytics, machine learning not only provides a glimpse into the future based on historical data but does so with a precision that redefines the boundaries of foresight.
Consider the ramifications in manufacturing, where predictive analytics powered by machine learning preemptively identifies potential equipment failures, averting costly downtime and streamlining production processes. This, however, is just the tip of the iceberg. The integration of neural networks, with their capacity for adaptive learning and mimicking cognitive functions, elevates machine learning to a level where it mirrors the cognitive faculties of the human brain. In doing so, it addresses challenges that were hitherto deemed insurmountable by conventional programming methods.
The practical applications of neural networks are as diverse as they are profound. In quality control, they enhance image recognition, ensuring that defects are identified with a precision that exceeds human capabilities. In autonomous systems, speech processing capabilities fueled by neural networks enable seamless human-machine interactions, fostering a new era of user-friendly interfaces. These applications exemplify how the synthesis of machine learning and engineering is not just about problem-solving but about redefining the possibilities within the realm of technology.
Imagine the implications for civil engineering where structural designs are optimized through machine learning-driven simulations, ensuring not just safety but efficiency in resource utilization. Picture the advancements in fluid dynamics research, where machine learning models simulate and predict the behavior of complex systems, revolutionizing our understanding of aerodynamics.
As we synthesize these advancements, it becomes evident that the collaboration between machine learning and engineering is not a mere augmentation of existing methodologies but a reimagining of what is possible. The future holds the promise of continued innovation and discovery as this symbiotic relationship propels us toward uncharted territories.
In conclusion, breaking down complex engineering problems with machine learning is not a singular accomplishment but a continuous journey marked by ongoing exploration and discovery. The impact is not confined to specific industries or niches; it resonates across the entire spectrum of engineering disciplines, from manufacturing and logistics to design and simulation. The narrative woven by machine learning in the intricate fabric of engineering problem-solving is dynamic, evolving, and limitless. As we navigate this uncharted territory, the collaborative dance between technology and engineering prowess promises not just incremental improvements but paradigm shifts that will define the technological landscape of the future.