Claim Your Discount Today
Ring in Christmas and New Year with a special treat from www.programminghomeworkhelp.com! Get 15% off on all programming assignments when you use the code PHHCNY15 for expert assistance. Don’t miss this festive offer—available for a limited time. Start your New Year with academic success and savings. Act now and save!
We Accept
- Understanding AI Challenges in Engineering
- Navigating the Complexities of AI Integration in Engineering Systems
- Compatibility Challenges - Navigating the Complex Interplay
- Data Integration Complexities - Synchronizing the Data Symphony
- Mastering Algorithmic Challenges in AI Engineering Assignments
- Balancing Precision and Efficiency - The Art of Algorithmic Equilibrium
- Handling Unstructured Data - Decoding the Language of Complexity
- Navigating the Ethical Landscape of AI in Engineering
- Addressing Bias in AI Algorithms - The Pursuit of Algorithmic Fairness
- Ensuring Responsible AI Implementation - Navigating the Societal Impact
- Optimizing Efficiency with Tools and Technologies in AI Engineering
- Machine Learning Libraries - Empowering Engineering Prowess
- Cloud Computing for Scalability - Unleashing Infinite Horizons
- Conclusion
In the swiftly advancing landscape of technology, Artificial Intelligence (AI) emerges as a transformative force within engineering. Its revolutionary potential extends from the optimization of processes to the facilitation of predictive analysis, fundamentally reshaping how we confront challenges in the engineering domain. This blog serves as an exploration into the intricate realm of AI challenges specific to engineering, aiming to provide pragmatic solutions for effectively navigating this dynamic field. By addressing the multifaceted complexities of AI integration, optimizing algorithms, navigating ethical considerations, and harnessing cutting-edge tools and technologies, engineers can unlock the full spectrum of possibilities that AI offers in their assignments. Whether you're looking to solve your Artificial Intelligence assignment or seeking ways to propel innovation, this blog provides insights and strategies to empower engineers in understanding and harnessing the transformative potential of AI in the ever-evolving landscape of engineering. As this field continues its rapid evolution, staying informed and adaptable becomes imperative for overcoming challenges and propelling innovation in AI engineering. Through a thoughtfully curated journey, this blog endeavors to empower engineers with the insights and strategies needed to not only understand but also harness the transformative potential of AI in the ever-evolving landscape of engineering.
Understanding AI Challenges in Engineering
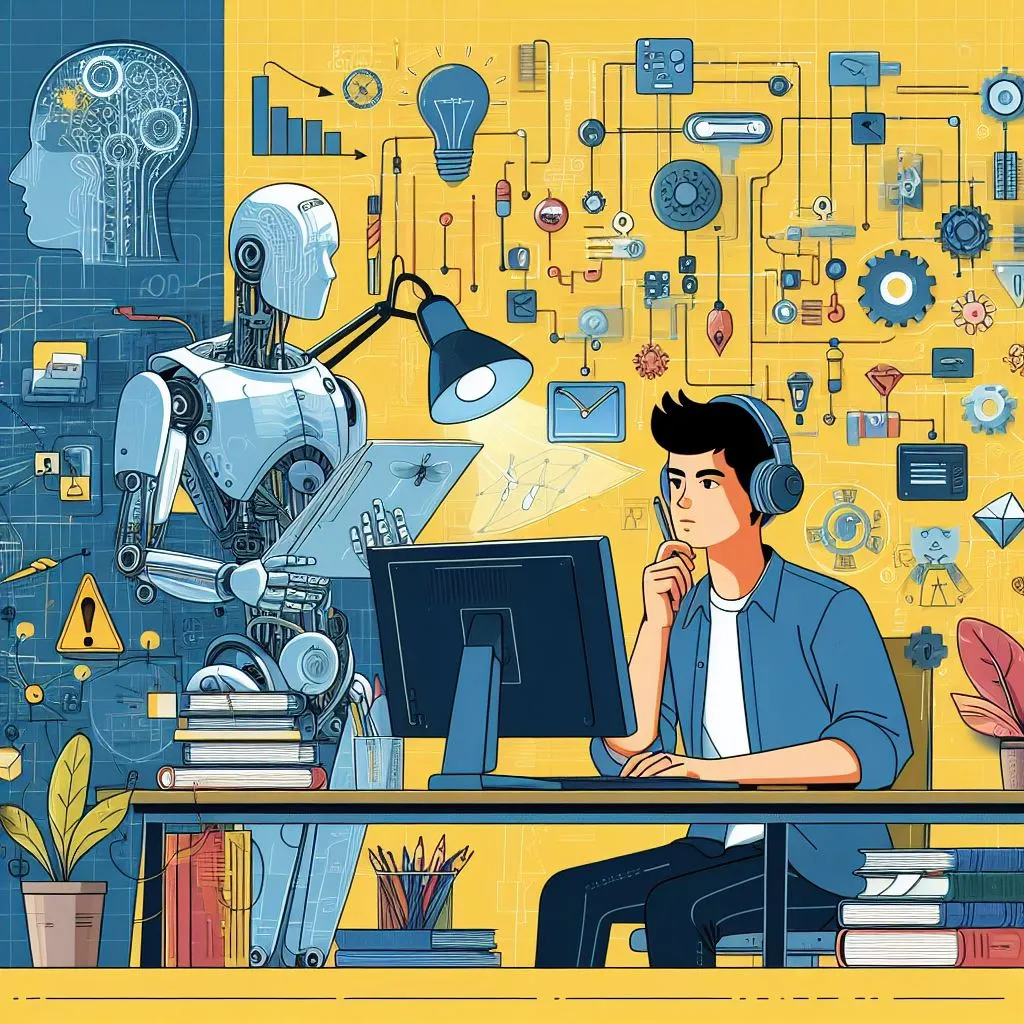
Delving into the intricate realm of AI challenges within the engineering domain requires a nuanced comprehension of multifaceted complexities. This section provides a detailed exploration of the hurdles engineers encounter when integrating AI into existing systems. From addressing compatibility issues that arise during integration to navigating the intricacies of data integration, understanding these challenges is fundamental for successful AI implementation. As engineering landscapes continue to evolve, the need to comprehend the unique hurdles posed by AI becomes paramount. This section serves as a comprehensive guide, offering insights and solutions to empower engineers in overcoming the complexity of AI integration in their assignments. By grasping the nuances encapsulated in these challenges, engineers can adopt a proactive stance, ensuring a seamless and effective integration of AI technologies into the dynamic field of engineering.
Navigating the Complexities of AI Integration in Engineering Systems
Embarking on the integration of AI within engineering systems unfolds a realm of distinctive challenges demanding meticulous navigation. This section encapsulates the intricate dynamics engineers face when seamlessly incorporating AI into existing frameworks. Whether contending with compatibility issues or unraveling the intricacies of data integration, engineers find themselves immersed in a complex landscape. The challenges posed by the amalgamation of AI into engineering systems are multifaceted, requiring a nuanced understanding and strategic solutions. This section offers a detailed exploration into these complexities, providing valuable insights to guide engineers in addressing and surmounting the hurdles encountered during the process of AI integration. By shedding light on the nuanced aspects of this journey, engineers can gain a deeper understanding of the challenges inherent in AI integration, fostering a more informed and effective approach to implementation within the realm of engineering systems.
Compatibility Challenges - Navigating the Complex Interplay
Compatibility challenges emerge as a nuanced facet of AI integration, where algorithms may not seamlessly align with existing engineering systems. This subsection delves deeper into the intricacies of this challenge, emphasizing the critical need for a nuanced understanding of system integration nuances. Engineers must navigate the complex interplay between AI algorithms and established systems, identifying potential points of friction and devising effective compatibility solutions. As technology evolves, the compatibility landscape transforms, requiring engineers to stay agile in their approach. This exploration extends beyond mere recognition of challenges, delving into proactive strategies to adapt and optimize systems for successful AI implementation. By dissecting compatibility challenges at a granular level, this section equips engineers with actionable insights, empowering them to navigate the evolving terrain of AI integration in engineering seamlessly.
Data Integration Complexities - Synchronizing the Data Symphony
In the realm of AI, effective implementation hinges on the orchestration of robust data integration. This subsection amplifies the focus on the complexities inherent in marrying AI algorithms with the vast troves of data generated in engineering processes. Beyond the surface-level acknowledgment of data challenges, engineers are guided through the intricate process of streamlining data integration. The challenges extend beyond storage issues to encompass processing bottlenecks and real-time access demands. Here, engineers delve into strategies that transcend conventional approaches, exploring innovative methods to synchronize the data symphony seamlessly. From adopting advanced data storage solutions to implementing real-time processing frameworks, this section provides a comprehensive guide to engineers seeking optimal AI performance through strategic and efficient data integration.
Mastering Algorithmic Challenges in AI Engineering Assignments
At the core of engineering assignments lies the pivotal task of crafting potent AI algorithms, a task laden with challenges. Engineers grapple with the delicate balance between efficiency and accuracy, a nuanced interplay that defines the success of AI endeavors. This section delves into the intricate landscape of algorithmic challenges that frequently confront engineers. From ensuring precision without compromising efficiency to addressing the complexities of handling unstructured data, the journey of algorithmic development is fraught with obstacles. Within this section, a comprehensive examination of these challenges is undertaken, providing engineers with practical solutions to navigate and conquer the intricacies of algorithmic complexities. By dissecting the common stumbling blocks and offering strategic insights, this segment empowers engineers to elevate their proficiency in crafting algorithms, thus fortifying their capabilities in the realm of AI engineering assignments.
Balancing Precision and Efficiency - The Art of Algorithmic Equilibrium
The perpetual challenge of achieving a delicate equilibrium between precision and efficiency in AI algorithms is a pivotal focus of this subsection. Engineers navigate the intricate terrain where algorithmic accuracy must not be sacrificed for the sake of processing speed and vice versa. Here, the exploration transcends the acknowledgment of the challenge itself, delving into the artistry of optimization. Techniques to strike the elusive balance are dissected, providing engineers with a toolkit of strategies to fine-tune algorithms effectively. From algorithmic pruning to parallel processing methodologies, this section delves into the nuanced realm of algorithmic equilibrium. Engineers are not only equipped with theoretical insights but are guided through practical implementations, fostering a holistic understanding of how to achieve optimal results without compromising on either precision or efficiency.
Handling Unstructured Data - Decoding the Language of Complexity
Engineering assignments often plunge into the intricate realm of unstructured data, be it images, texts, or sensor readings. This subsection elevates the discussion beyond the recognition of unstructured data challenges, immersing engineers in the art of decoding the language of complexity. Successfully processing and extracting meaningful insights from unstructured data become pivotal components of AI implementation in engineering. Through a detailed exploration of advanced techniques such as natural language processing (NLP), computer vision, and sensor fusion, engineers gain not only theoretical insights but practical strategies to unravel the richness of unstructured data. By navigating the complexities inherent in these datasets, engineers can harness the full potential of AI in engineering assignments, transcending traditional boundaries and achieving transformative results.
Navigating the Ethical Landscape of AI in Engineering
The integration of AI in engineering introduces a complex tapestry of ethical considerations, demanding a conscientious exploration. This section unravels the myriad ethical challenges that surface, ranging from the inadvertent biases embedded in algorithms to the overarching responsibility associated with AI deployment. Engineers find themselves at the intersection of technological innovation and ethical dilemmas, navigating a landscape that requires a delicate balance. Delving into the intricacies of these ethical considerations, this segment serves as a guide for engineers seeking a nuanced understanding and responsible approach. By shedding light on the potential biases within algorithms and emphasizing the need for the ethical deployment of AI, this section equips engineers with the insights and guidance necessary to navigate the ethical dimensions of AI in engineering conscientiously.
Addressing Bias in AI Algorithms - The Pursuit of Algorithmic Fairness
The pressing concern of bias in AI algorithms takes center stage in this subsection, urging engineers to confront the potential repercussions of unfair outcomes. Beyond mere acknowledgment, engineers delve into the proactive realm of identifying and mitigating bias within their AI systems. The exploration extends beyond theoretical understanding to practical strategies, guiding engineers through the intricate process of pursuing algorithmic fairness. From algorithmic auditing to the incorporation of diverse datasets, this section equips engineers with a comprehensive toolkit to address bias systematically. By adopting these strategies, engineers not only ensure the fairness of their AI systems but also contribute to the broader conversation on mitigating bias in technological solutions, fostering a more inclusive and equitable landscape.
Ensuring Responsible AI Implementation - Navigating the Societal Impact
The critical facet of responsible AI implementation unfolds as engineers contemplate the potential societal impact of their engineering solutions in this subsection. Moving beyond a surface-level understanding, engineers explore the nuanced layers of ethical considerations embedded in AI assignments. This exploration extends beyond the immediate technical realm, delving into the broader societal implications of AI deployment. A framework for responsible AI engineering emerges, guiding engineers through the intricate process of aligning technological advancements with ethical imperatives. From stakeholder engagement to impact assessments, this section empowers engineers to navigate the complexities of responsible AI implementation, ensuring that technological progress aligns harmoniously with societal well-being. Through a conscientious approach, engineers emerge not just as technical architects but as stewards of responsible and ethical AI deployment, shaping a future where innovation is synonymous with societal benefit.
Optimizing Efficiency with Tools and Technologies in AI Engineering
Within the dynamic sphere of artificial intelligence, engineers are presented with a diverse array of tools and technologies poised to streamline their tasks. This section serves as a gateway to the expansive toolkit available in the rapidly advancing field of AI. Offering an introduction to key tools and technologies, engineers can explore innovative solutions that significantly enhance the efficiency of their AI engineering assignments. From machine learning libraries such as TensorFlow and PyTorch to the scalability benefits offered by cloud computing, this segment provides a comprehensive overview, enabling engineers to make informed decisions regarding the integration of cutting-edge tools into their workflows. As the landscape of AI technology evolves, staying abreast of these instrumental tools becomes essential, ensuring that engineers are well-equipped to navigate the complexities of AI engineering with precision and efficacy.
Machine Learning Libraries - Empowering Engineering Prowess
This subsection delves into the expansive realm of machine learning libraries, where powerhouse tools like TensorFlow and PyTorch emerge as catalysts for AI model development. Engineers are not merely introduced to these libraries but guided through an exploration of their capabilities. The discussion transcends the theoretical to the practical, equipping engineers with insights on how to leverage these libraries effectively in their assignments. From understanding the nuances of algorithm implementation to harnessing the full potential of pre-built models, engineers navigate a landscape where machine learning libraries become not just tools but allies in the quest for engineering prowess. Through this exploration, engineers gain a comprehensive understanding of the capabilities embedded in these libraries, transforming them into adept architects of AI models poised for transformative impact.
Cloud Computing for Scalability - Unleashing Infinite Horizons
The subsection on cloud computing unfolds as a beacon for engineers seeking scalability and flexibility in their AI engineering tasks. Cloud computing, as a paradigm, is not merely introduced but dissected for its myriad benefits in handling the computational demands of AI algorithms. Engineers are guided through a journey where the scalability and flexibility offered by cloud platforms become integral components of their AI strategies. From harnessing the power of distributed computing to seamlessly scaling resources based on algorithmic requirements, this section provides a roadmap for engineers to navigate the expansive landscape of cloud computing. By understanding the symbiotic relationship between AI and cloud platforms, engineers unlock the door to infinite horizons, where computational resources align seamlessly with the evolving demands of AI engineering tasks.
Conclusion
In conclusion, tackling the intricate challenges posed by AI in engineering necessitates a comprehensive strategy encompassing technical, ethical, and practical dimensions. Engineers can harness the transformative power of AI in their assignments by delving into the nuances of integration, fine-tuning algorithms for efficiency, and navigating ethical considerations to ensure responsible implementation. Furthermore, the adept utilization of state-of-the-art tools and technologies proves instrumental in unlocking the full potential of AI. As the landscape of AI engineering evolves, maintaining a constant commitment to staying informed and adaptable becomes paramount. This adaptability is not merely a response to challenges but a proactive stance towards driving innovation within the dynamic sphere of AI engineering. Through a holistic and strategic approach, engineers can not only overcome existing challenges but also pioneer new frontiers, contributing to the ongoing evolution of AI within the engineering domain.