Claim Your Offer
New semester, new challenges—but don’t stress, we’ve got your back! Get expert programming assignment help and breeze through Python, Java, C++, and more with ease. For a limited time, enjoy 10% OFF on all programming assignments with the Spring Special Discount! Just use code SPRING10OFF at checkout! Why stress over deadlines when you can score high effortlessly? Grab this exclusive offer now and make this semester your best one yet!
We Accept
- Understanding Neural Networks: A Primer
- A. Basics of Neural Network Architecture
- Neural Networks in Machine Learning Assignments
- A. Pattern Recognition and Image Classification
- B. Natural Language Processing (NLP) Assignments
- Real-world Applications: Bridging Theory and Practice
- A. Healthcare: Diagnosis and Predictive Analysis
- B. Autonomous Systems: Robotics and Self-driving Vehicles
- Challenges and Ethical Considerations
- A. Overfitting and Model Optimization Challenges
- B. Ethical Use of AI: A Call to Responsibility
- Future Trends: Preparing Students for Tomorrow's Challenges
- A. Reinforcement Learning: Beyond Supervised and Unsupervised Learning
- B. Explainable AI: Bridging the Gap Between AI and Humans
- Conclusion
as a transformative powerhouse, reshaping our approach to intricate problem-solving. For engineering students, delving into the intricacies of Neural Networks transcends mere academic necessity; it evolves into a pivotal skill shaping their future in the tech industry. This blog embarks on a comprehensive exploration of the fundamental role played by Neural Networks in the realm of AI, shedding light on their profound significance within engineering student assignments. From unraveling the basics of Neural Network architecture to navigating real-world applications and addressing ethical considerations, the journey through this blog aims to equip students with the essential skills demanded by the dynamic intersection of technology and academia. As we unravel the layers of Neural Networks, we aim to empower engineering students, not just for current academic requirements but to cultivate a proficiency that positions them as adept contributors in the unfolding narrative of AI and technology. Whether you're a student seeking assistance with your AI assignment or an enthusiast looking to deepen your understanding of Neural Networks, this exploration is designed to provide valuable insights and support for your academic journey.
Understanding Neural Networks: A Primer
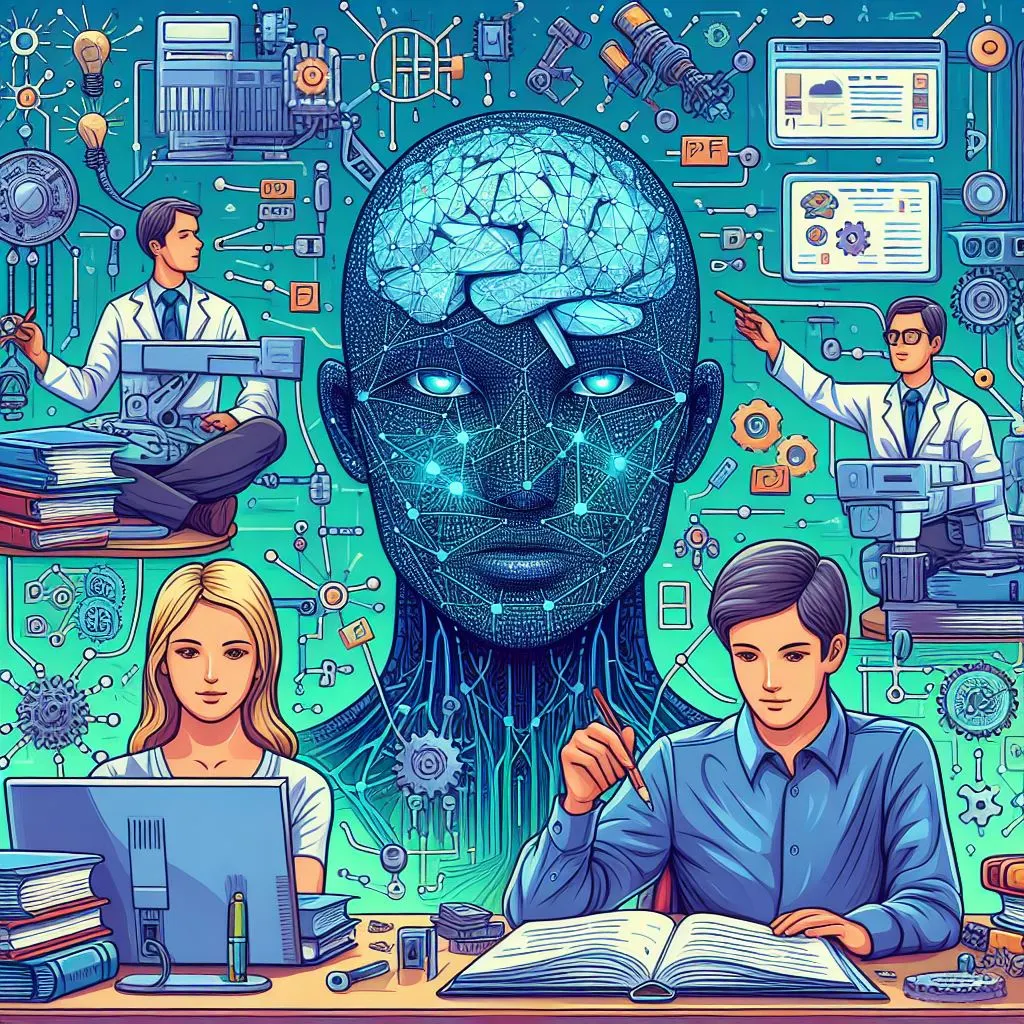
In the realm of AI, grasping the fundamentals of Neural Networks is paramount. Before exploring their role in assignments, it is essential to establish a solid foundation by comprehending what Neural Networks truly are. These networks, akin to the intricacies of the human brain, operate through a set of algorithms meticulously designed for pattern recognition. Characterized by layers of interconnected nodes, Neural Networks excel in diverse tasks such as image and speech recognition, natural language processing, and beyond. This primer sets the stage for a nuanced exploration, providing readers with the necessary insights into the architectural intricacies that underpin the functionality of Neural Networks and their multifaceted applications in the expansive field of artificial intelligence.
A. Basics of Neural Network Architecture
To truly appreciate the role of Neural Networks in AI assignments, a deep understanding of their fundamental architecture is essential. The intricacies of a Neural Network lie in its layered structure, encompassing input layers, hidden layers, and output layers. Each layer is not just a mere component but a critical actor in the complex orchestration of processing and transforming information. The input layers receive and interpret data, serving as the gateway for information to enter the network. As this data traverses through hidden layers, intricate computations occur, enabling the network to recognize patterns and extract meaningful features. The final output layers synthesize the processed information into actionable insights. This architectural journey is akin to peeling back the layers of a technological onion, revealing the nuanced mechanisms that make Neural Networks a potent force in the realm of artificial intelligence assignments. By comprehending this intricate architecture, students lay the groundwork for harnessing the full potential of Neural Networks in their academic and future professional endeavors.
Neural Networks in Machine Learning Assignments
In the evolving landscape of AI integration, machine learning assignments have become a focal point for engineering students, frequently incorporating tasks centered around Neural Networks. This section delves into the symbiotic relationship between Neural Networks and machine learning assignments, shedding light on the practical applications and skills cultivated by students. As AI permeates diverse fields, understanding the pivotal role Neural Networks play in machine learning tasks becomes crucial for aspiring engineers. The exploration within this segment aims to unveil the dynamic intersection of theoretical knowledge and practical application, offering insights into how Neural Networks serve as the backbone of machine learning assignments and contribute to the skill set essential for navigating the intricacies of contemporary technology.
A. Pattern Recognition and Image Classification
In the expansive landscape of Neural Networks, image classification emerges as a cornerstone application, and its mastery becomes a focal point in student assignments. Tasks in this domain propel students beyond theoretical understanding to practical implementation, challenging them to intricately design and optimize Neural Networks for precise image classification. The assignments serve as an immersive experience, fostering proficiency not only in Neural Networks but also in the broader realms of image processing and intricate pattern recognition. Through this hands-on exploration, students not only decode the complexities of image classification but also cultivate a skill set that extends into diverse fields reliant on visual data interpretation.
B. Natural Language Processing (NLP) Assignments
The ascendancy of chatbots and language models positions Natural Language Processing (NLP) assignments at the forefront of engineering curricula. Within this domain, Neural Networks play a pivotal role in multifaceted tasks such as sentiment analysis, text summarization, and language translation. Assignments in NLP propel students into the heart of language understanding, requiring them to implement and optimize Neural Networks for nuanced linguistic tasks. Engaging in these assignments provides students with invaluable hands-on experience, not only refining their proficiency in Neural Networks but also deepening their understanding of the intricate interplay between machines and human language. This immersive journey equips students with skills vital in an era where effective human-computer communication is paramount.
Real-world Applications: Bridging Theory and Practice
Venturing beyond the theoretical realm, this section illuminates the tangible impact of Neural Networks in real-world scenarios, providing engineering students with a bridge between theory and practice. By exploring applications that extend beyond the boundaries of assignments, students gain valuable insights into how their knowledge of Neural Networks translates into transformative solutions across various industries. This segment serves as a conduit for understanding the direct relevance and practical implications of Neural Network expertise, preparing students to navigate the complexities of real-world challenges and contribute meaningfully to sectors where AI applications are increasingly becoming integral.
A. Healthcare: Diagnosis and Predictive Analysis
The impact of Neural Networks in healthcare transcends theoretical prowess, extending into tangible advancements in diagnosis and predictive analysis. Assignments in this domain immerse engineering students in the cutting-edge applications of Neural Networks, where they delve into the intricate world of medical diagnostics. Through these assignments, students actively contribute to the evolution of healthcare technology, gaining insights into how Neural Networks discern patterns in medical data to aid in disease diagnosis and prognosis. This hands-on experience not only fortifies their understanding of Neural Networks but also instills a profound sense of responsibility as they witness firsthand the potential of AI to revolutionize healthcare outcomes.
B. Autonomous Systems: Robotics and Self-driving Vehicles
The fusion of Neural Networks with autonomous systems propels engineering students into the future of robotics and transportation. Assignments in this realm serve as gateways to understanding and implementing Neural Networks in the complex decision-making processes of robots and self-driving vehicles. Through these assignments, students grapple with real-world challenges, devising solutions that enable autonomous systems to navigate environments and make informed decisions. This practical engagement equips students with a forward-looking perspective, preparing them for the technological landscape where Neural Networks play a pivotal role in shaping the future of robotics, transportation, and the broader field of autonomous systems. The assignments become a conduit for students to actively contribute to the ongoing revolution in these transformative domains.
Challenges and Ethical Considerations
Navigating the intricacies of Neural Networks goes beyond mastering technicalities; it involves confronting challenges and ethical quandaries that influence an engineer's approach. This section delves into the hurdles encountered by engineering students as they delve into the world of Neural Networks, emphasizing the importance of addressing common challenges such as overfitting and model optimization. Furthermore, it prompts students to reflect on the ethical dimensions inherent in their work, fostering a heightened sense of responsibility in the development and application of AI. By acknowledging and addressing these challenges head-on, students gain a holistic understanding of Neural Networks, preparing them not only to surmount technical obstacles but also to navigate the ethical complexities that come with wielding the power of artificial intelligence.
A. Overfitting and Model Optimization Challenges
In the realm of Neural Networks, assignments frequently thrust engineering students into the challenging terrain of overfitting and model optimization. These assignments are not mere exercises; they are gateways to developing problem-solving acumen crucial for real-world applications. Overfitting issues demand students to scrutinize and fine-tune models, ensuring optimal performance. Through these challenges, students cultivate a profound understanding of the delicate balance between model complexity and generalizability. The assignments become more than academic exercises; they transform into laboratories where students hone skills essential for tackling the nuanced challenges prevalent in deploying Neural Networks across diverse applications.
B. Ethical Use of AI: A Call to Responsibility
As engineering students embark on Neural Network assignments, they enter the realm of ethical considerations, a domain equally vital as technical proficiency. This section serves as a poignant reminder of the responsibilities accompanying the development and application of AI. Beyond the algorithms and computations, students are prompted to reflect on the ethical implications inherent in their solutions. From addressing bias in data to understanding the societal impact of AI, this section underscores the imperative of responsible AI development. The assignments become a platform for students to not only master the intricacies of Neural Networks but also to cultivate an ethical mindset, preparing them to navigate the complex landscape where technology intersects with human values and societal well-being.
Future Trends: Preparing Students for Tomorrow's Challenges
As the field of AI continues its rapid evolution, anticipating and adapting to future trends becomes imperative. This section serves as a compass, guiding engineering students through the exploration of emerging trends within Neural Networks. By investigating how these trends intertwine with assignments, students gain a forward-thinking perspective, ensuring they are not merely meeting current requirements but are well-prepared to tackle the challenges of tomorrow. From the burgeoning realm of reinforcement learning to the imperative of Explainable AI, this segment navigates the uncharted territories, equipping students with the foresight and skills required to innovate and lead in an ever-changing AI landscape. In essence, it prepares them to not only embrace current advancements but also to proactively shape the trajectory of Neural Networks in the dynamic future of artificial intelligence.
A. Reinforcement Learning: Beyond Supervised and Unsupervised Learning
In the trajectory of AI, the future unfolds with the paradigm of reinforcement learning, a realm where Neural Networks evolve through trial and error. Assignments that usher students into the intricacies of reinforcement learning concepts serve as visionary portals, opening pathways to innovative problem-solving in AI. These assignments are not just academic exercises; they are the stepping stones toward a future where AI systems dynamically adapt and improve through continuous interaction with their environments. By immersing themselves in reinforcement learning assignments, students traverse the forefront of AI evolution, acquiring skills that transcend the traditional boundaries of supervised and unsupervised learning, thus preparing them to usher in a new era of adaptive, learning machines.
B. Explainable AI: Bridging the Gap Between AI and Humans
The evolving landscape of AI introduces a critical imperative—Explainable AI. Understanding the decisions orchestrated by Neural Networks is not just a technical nuance but a societal necessity. Future assignments could center on Explainable AI, guiding students to develop models that transcend the enigmatic nature of AI decision-making. In these assignments, students delve into the complex realm of transparent insights, aiming to bridge the gap between AI and human comprehension. The focus shifts from mere model accuracy to fostering a profound understanding of the decision-making processes, ensuring that AI applications align with human values and ethical considerations. By engaging in Explainable AI assignments, students become architects of a future where the decisions of Neural Networks are not opaque but are accessible, interpretable, and ethically aligned with human expectations.
Conclusion
In conclusion, the pivotal role of Neural Networks in AI assignments for engineering students cannot be overstated. The journey from mastering the fundamentals to addressing real-world challenges and ethical considerations ensures that students are well-equipped with the requisite skills demanded by the contemporary technological landscape. This exploration of Neural Networks not only fulfills current academic requirements but also serves as a springboard for students to lead innovations in the ever-evolving AI domain. Looking ahead, the integration of emerging trends becomes imperative in shaping a cohort of engineers who are not merely adept at meeting present demands but are prepared to proactively shape the future of AI. In essence, as we conclude our exploration of Neural Networks, it becomes evident that the knowledge and skills acquired by engineering students in this domain are instrumental in empowering them for a future that is undeniably AI-driven and brimming with opportunities for pioneering advancements.