Claim Your Discount Today
Ring in Christmas and New Year with a special treat from www.programminghomeworkhelp.com! Get 15% off on all programming assignments when you use the code PHHCNY15 for expert assistance. Don’t miss this festive offer—available for a limited time. Start your New Year with academic success and savings. Act now and save!
We Accept
- Understanding Epidemiological Modeling
- 1. Deterministic Models:
- 2. Stochastic Models:
- The Role of NetLogo in Health Sciences Assignments
- 1. Agent-Based Modeling:
- 2. Customizable Parameters:
- 3. Visualization Tools:
- Applications of NetLogo in Public Health
- 1. Modeling Transmission Dynamics:
- 2. Intervention Strategies:
- 3. Impact Assessment:
- 4. Resource Allocation:
- Benefits and Limitations of Using NetLogo in Health Sciences Assignments
- 1. User-Friendly Interface:
- 2. Educational Value:
- 3. Collaborative Learning:
- 1. Simplistic Assumptions:
- 2. Computational Constraints:
- 3. Data Sensitivity:
- Case Studies: Real-world Applications of NetLogo in Public Health
- 1. COVID-19 Pandemic Analysis:
- 2. Vector-Borne Diseases:
- Conclusion
Epidemiological modeling stands as a pivotal cornerstone in deciphering the intricate dynamics of disease transmission, control, and prevention within diverse populations. As the realm of public health continuously evolves, technological advancements and computational innovations have seamlessly integrated sophisticated software applications, notably NetLogo, into health sciences curricula and research endeavors. This integration heralds a transformative approach, allowing practitioners, educators, and students alike to simulate and analyze complex epidemiological scenarios with unparalleled precision and depth. By leveraging the capabilities of NetLogo, health sciences assignments transcend traditional boundaries, fostering an enriched learning environment that emphasizes hands-on exploration, critical thinking, and evidence-based decision-making. Furthermore, the symbiotic relationship between epidemiological modeling and NetLogo facilitates nuanced insights into disease spread mechanisms, intervention efficacy, and public health policy formulation. In essence, this convergence of technology and epidemiology not only underscores the paramount importance of data-driven strategies in combating global health challenges but also paves the way for future innovations, collaborations, and advancements in the ever-evolving landscape of public health. If you need help with your NetLogo assignment, leveraging the capabilities of NetLogo for epidemiological modeling can provide invaluable insights and enhance your understanding of disease transmission dynamics and public health interventions.
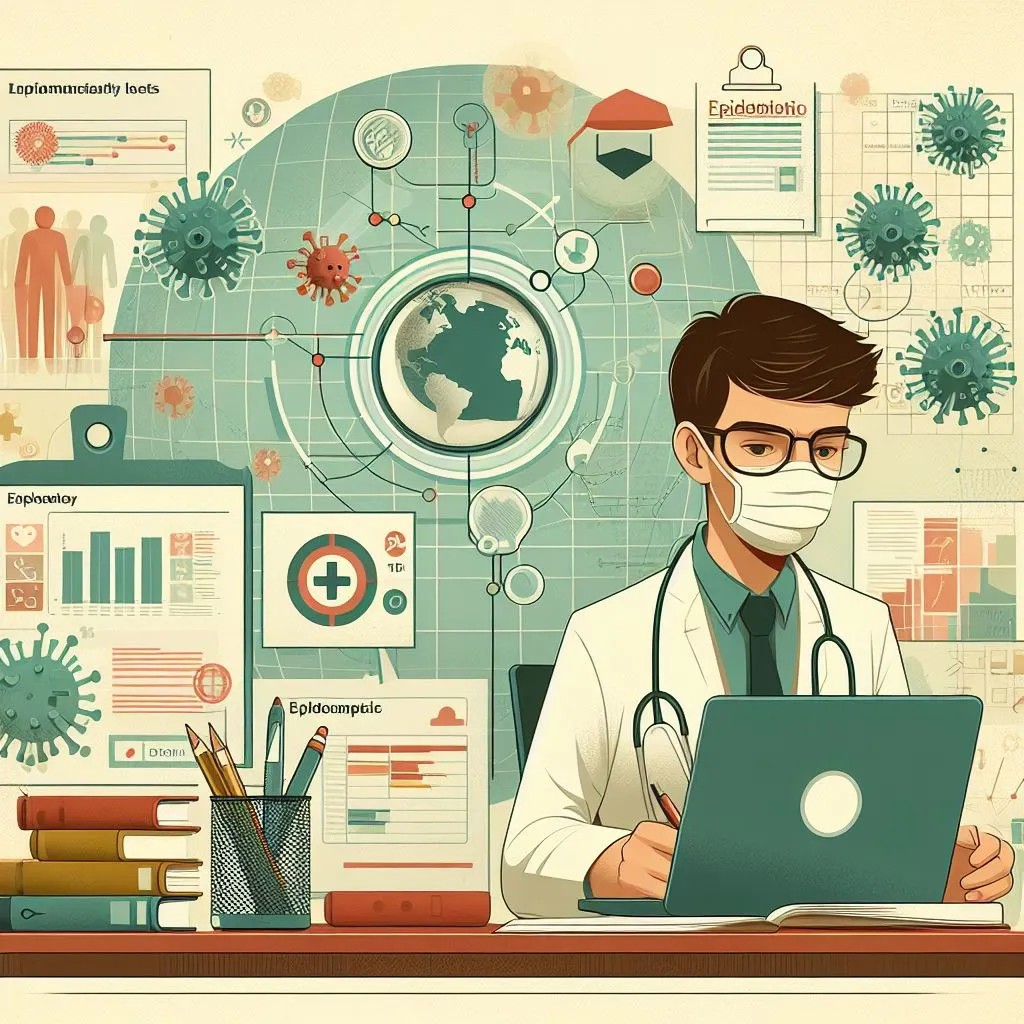
Understanding Epidemiological Modeling
Epidemiological modeling intricately weaves mathematical and computational frameworks to elucidate the intricate dynamics of disease propagation within diverse populations. Serving as a cornerstone in public health endeavors, this modeling approach empowers professionals with predictive insights, enabling proactive measures against disease spread. By leveraging advanced algorithms and data-driven methodologies, epidemiological modeling facilitates rigorous evaluation of intervention strategies, ensuring their efficacy and relevance in real-world contexts. Furthermore, this analytical approach transcends traditional boundaries, fostering the design and implementation of targeted control strategies tailored to mitigate disease risks effectively. In essence, understanding the nuances of epidemiological modeling not only amplifies our capacity to anticipate and address public health challenges but also underscores its pivotal role in shaping resilient, adaptive, and evidence-based healthcare systems globally.
Types of Epidemiological Models
1. Deterministic Models:
Deterministic models operate on the premise of fixed parameters and assumptions, providing a structured framework to predict outcomes without factoring in randomness. These models offer a streamlined approach, enabling researchers and practitioners to develop concise projections and strategies based on predefined variables. However, it's crucial to acknowledge that deterministic models may oversimplify complex epidemiological dynamics, potentially leading to deviations from real-world scenarios. While they offer valuable insights, their deterministic nature limits their ability to account for inherent uncertainties and variability within disease spread patterns.
2. Stochastic Models:
In contrast, stochastic models embrace randomness and variability, striving to emulate realistic scenarios by incorporating uncertainties inherent in disease spread dynamics. By simulating fluctuations and unpredictabilities, these models provide a more comprehensive and nuanced perspective, facilitating a deeper understanding of epidemic trajectories and potential outcomes. This approach resonates with the multifaceted nature of epidemiological phenomena, emphasizing the importance of probabilistic frameworks and adaptive strategies. However, harnessing stochastic models necessitates meticulous calibration, validation, and interpretation to ensure accuracy, reliability, and relevance in guiding public health interventions and policy formulations.
The Role of NetLogo in Health Sciences Assignments
NetLogo stands out as a prominent agent-based modeling platform, renowned for its user-friendly interface and robust capabilities in simulating diverse epidemiological scenarios. Catering to the intricate demands of health sciences assignments, its versatility and adaptability resonate with educators, students, and researchers alike. By seamlessly integrating complex computational algorithms with intuitive design features, NetLogo empowers stakeholders to explore, analyze, and navigate multifaceted health-related challenges with unparalleled precision and insight. This transformative tool transcends conventional boundaries, fostering an enriched learning environment that prioritizes hands-on exploration, critical thinking, and evidence-based inquiry. In essence, NetLogo's pivotal role in health sciences assignments underscores its intrinsic value in advancing educational outcomes, promoting collaborative research endeavors, and cultivating a new generation of public health professionals equipped with cutting-edge modeling and analytical skills.
Key Features of NetLogo
1. Agent-Based Modeling:
NetLogo's agent-based modeling capability stands as a hallmark feature, enabling the simulation of individual agents, such as people or entities, and their intricate interactions within a meticulously defined environment. By capturing micro-level dynamics and emergent behaviors, this approach fosters a granular understanding of complex systems, elucidating patterns, relationships, and interdependencies. This nuanced simulation framework resonates with real-world complexities, offering insights into individual behaviors, group dynamics, and systemic interactions. However, harnessing agent-based modeling requires a comprehensive understanding of agent attributes, interactions, and environmental factors to ensure accurate representation and interpretation of simulation outcomes.
2. Customizable Parameters:
Another distinguishing feature of NetLogo lies in its ability to facilitate customizable parameters, empowering users to tailor model specifications, including transmission rates, population sizes, intervention strategies, and myriad variables. This adaptability fosters flexibility, enabling stakeholders to explore diverse scenarios, test hypotheses, and evaluate strategies under varying conditions. This iterative approach resonates with dynamic public health landscapes, promoting innovation, optimization, and adaptive responses. Nonetheless, customization mandates rigorous calibration, validation, and sensitivity analyses to ensure model robustness, reliability, and applicability across diverse epidemiological contexts.
3. Visualization Tools:
NetLogo's visualization tools encompass a pivotal feature, offering graphical representations of simulation outcomes that transcend numerical data, fostering enhanced data interpretation, analysis, and communication. By translating complex datasets into intuitive visualizations, these tools facilitate stakeholder engagement, knowledge dissemination, and evidence-based decision-making processes. This visual-centric approach resonates with diverse audiences, promoting transparency, accessibility, and collaborative exploration of epidemiological phenomena. However, leveraging visualization tools necessitates discernment, ensuring clarity, accuracy, and relevance in portraying simulation outcomes while mitigating potential misinterpretations or biases inherent in graphical representations.
Applications of NetLogo in Public Health
NetLogo's expansive applicability resonates across multifaceted domains within the realm of public health, serving as a catalyst for innovation and transformative solutions. Its intrinsic versatility empowers researchers and practitioners to navigate and address complex epidemiological challenges with heightened precision and efficacy. By harnessing its computational prowess and analytical capabilities, stakeholders can dissect intricate disease dynamics, evaluate intervention strategies, and formulate evidence-based policies tailored to diverse populations and contexts. This robust platform transcends traditional methodologies, fostering collaborative endeavors that prioritize data-driven insights, proactive risk mitigation, and holistic approaches to enhancing population health outcomes. In essence, NetLogo's pivotal role in public health underscores its significance as an indispensable tool, driving advancements, fostering resilience, and shaping a proactive landscape that prioritizes innovation, collaboration, and excellence in addressing global health challenges.
Disease Outbreak Analysis
1. Modeling Transmission Dynamics:
Delving into disease outbreak analysis necessitates a robust understanding of transmission dynamics, where modeling serves as a cornerstone in simulating disease spread predicated on intricate factors like population interactions, contact rates, and transmission modes. This comprehensive approach facilitates the elucidation of transmission pathways, hotspot identification, and vulnerability assessments, enabling stakeholders to proactively devise targeted interventions. Additionally, integrating real-time data, epidemiological insights, and contextual factors enhances model fidelity, fostering a nuanced understanding of epidemic trajectories, potential escalation patterns, and intervention efficacy. Nevertheless, modeling transmission dynamics mandates continuous refinement, validation, and adaptation to capture evolving epidemiological dynamics, population behaviors, and environmental factors effectively.
2. Intervention Strategies:
Analyzing intervention strategies within disease outbreak contexts entails evaluating the effectiveness of multifaceted control measures, encompassing vaccination campaigns, social distancing protocols, quarantine initiatives, and other pivotal interventions. This evaluative approach fosters evidence-based decision-making, resource allocation optimization, and proactive risk mitigation, emphasizing iterative strategy refinement based on real-world outcomes, epidemiological insights, and stakeholder feedback. Furthermore, leveraging modeling frameworks enables stakeholders to simulate diverse intervention scenarios, assess potential outcomes, and prioritize interventions based on efficacy, feasibility, and impact. However, navigating intervention strategies necessitates a holistic perspective, encompassing logistical considerations, ethical implications, societal acceptance, and adaptive responses to ensure alignment with evolving public health priorities, challenges, and dynamics.
3. Impact Assessment:
Health policy evaluation necessitates a meticulous impact assessment, encompassing the analysis of potential outcomes of public health policies and interventions on disease prevalence, mortality rates, and broader societal determinants of health. This evaluative framework facilitates the identification of policy effectiveness, unintended consequences, and areas necessitating strategic refinement or recalibration. By integrating epidemiological modeling, statistical analyses, and stakeholder feedback, impact assessment fosters evidence-based policymaking, transparency, and accountability. Moreover, evaluating policy ramifications across diverse population subgroups, geographical regions, and temporal dimensions enhances understanding, fosters equity considerations, and informs targeted interventions to address disparities effectively. Nonetheless, conducting impact assessments mandates rigorous methodologies, comprehensive data integration, and contextual sensitivity to ensure validity, reliability, and relevance in guiding policy formulation, implementation, and evaluation.
4. Resource Allocation:
Optimizing resource allocation stands as a pivotal component within health policy evaluation, emphasizing strategic distribution and utilization of critical resources, including healthcare facilities, personnel, supplies, and technological assets. By harnessing modeling predictions, stakeholders can forecast resource demands, assess supply-chain dynamics, and prioritize allocations based on epidemiological trends, population needs, and intervention priorities. This data-driven approach fosters efficiency, resilience, and responsiveness within healthcare systems, promoting equitable access, quality care delivery, and proactive risk mitigation. Furthermore, integrating multi-criteria decision analysis, cost-effectiveness evaluations, and ethical considerations enhances resource allocation strategies, fostering sustainability, stakeholder engagement, and alignment with broader health policy objectives. However, navigating resource allocation complexities necessitates continuous monitoring, evaluation, and adaptation to address evolving epidemiological dynamics, technological advancements, and societal priorities effectively.
Benefits and Limitations of Using NetLogo in Health Sciences Assignments
NetLogo's integration into health sciences assignments brings forth a plethora of advantages, yet concurrently necessitates a nuanced understanding of its inherent limitations and challenges within specific epidemiological contexts. Recognizing its multifaceted benefits enables stakeholders to leverage its computational capabilities, fostering enriched learning experiences, innovative research endeavors, and evidence-based decision-making processes. However, an astute awareness of its limitations, ranging from simplifications inherent in modeling assumptions to computational constraints, underscores the importance of critical evaluation, validation, and contextual applicability. Balancing the nuanced interplay between NetLogo's strengths and limitations facilitates a comprehensive approach, ensuring that its utilization aligns with educational objectives, research goals, and the intricacies of real-world public health scenarios. In essence, navigating the landscape of NetLogo in health sciences assignments mandates a balanced perspective that harnesses its potential while critically evaluating its constraints, thereby fostering a holistic and informed approach to addressing complex epidemiological challenges.
Benefits
1. User-Friendly Interface:
One of the standout benefits of utilizing NetLogo lies in its intuitive and user-friendly interface, designed to accommodate individuals with diverse expertise levels, ranging from novice learners to seasoned researchers. This accessibility fosters inclusivity, empowerment, and engagement, enabling stakeholders to navigate modeling complexities, customize parameters, and interpret outcomes with ease. By minimizing technical barriers and streamlining workflows, the user-friendly interface catalyzes innovation, exploration, and iterative learning, positioning NetLogo as a versatile tool within academic, research, and professional settings. However, leveraging this interface effectively necessitates foundational knowledge, continuous skill development, and contextual understanding to maximize its potential and applicability across diverse epidemiological contexts and research domains.
2. Educational Value:
NetLogo's educational value transcends traditional pedagogical approaches, fostering experiential learning, critical thinking, and conceptual mastery through hands-on simulation and analysis. By immersing students and researchers in simulated epidemiological scenarios, this interactive platform facilitates the exploration of complex concepts, dynamics, and real-world applications. This experiential learning paradigm cultivates a deeper understanding of epidemiological principles, modeling methodologies, and public health challenges, nurturing a new generation of informed, adaptive, and innovative professionals. Moreover, integrating NetLogo within educational curricula promotes interdisciplinary learning, problem-solving skills, and collaborative engagement, preparing individuals to address evolving health landscapes, societal needs, and global challenges with competence and confidence.
3. Collaborative Learning:
NetLogo's collaborative learning features resonate with the evolving landscape of education and research, fostering group assignments, research projects, and knowledge-sharing initiatives that prioritize collaboration, diversity, and inclusivity. By facilitating seamless communication, data sharing, and collaborative exploration, this platform empowers teams to leverage collective expertise, perspectives, and resources, enhancing creativity, innovation, and problem-solving capacities. Furthermore, fostering a collaborative learning environment cultivates interpersonal skills, teamwork dynamics, and leadership competencies, preparing individuals to navigate complex public health challenges, interdisciplinary collaborations, and stakeholder engagements effectively. However, harnessing collaborative learning opportunities within NetLogo mandates fostering a culture of respect, inclusivity, and shared accountability, ensuring equitable contributions, diverse perspectives, and collective success in addressing multifaceted health-related challenges and opportunities.
Limitations
1. Simplistic Assumptions:
One of the primary limitations associated with NetLogo lies in its inherent tendency to employ simplistic assumptions when modeling complex epidemiological dynamics. This oversimplification may inadvertently overlook nuanced interactions, variables, and contingencies inherent within real-world scenarios, potentially compromising model accuracy, reliability, and validity. Consequently, reliance on overly simplistic assumptions necessitates vigilant scrutiny, critical evaluation, and contextual adaptation to ensure alignment with specific epidemiological contexts, research objectives, and policy implications. Furthermore, mitigating the impact of these limitations mandates integrating complementary methodologies, data sources, and analytical techniques to foster a more comprehensive, nuanced, and adaptive modeling approach that resonates with evolving public health challenges and complexities.
2. Computational Constraints:
Navigating NetLogo's computational constraints remains a significant consideration, particularly when modeling intricate scenarios, diverse populations, or multifaceted interventions that demand extensive computational resources, time, and expertise. Depending on model complexity, simulation parameters, and technological infrastructures, stakeholders may encounter challenges related to performance optimization, scalability, and responsiveness, potentially hindering real-time analysis, iterative refinement, and dynamic adaptation. Thus, addressing computational constraints necessitates leveraging advanced computational techniques, cloud-based resources, and collaborative platforms to enhance efficiency, reliability, and scalability while mitigating potential bottlenecks, limitations, and operational challenges associated with NetLogo's computational framework.
3. Data Sensitivity:
NetLogo's reliance on accurate data inputs, encompassing population demographics, disease characteristics, intervention effects, and contextual variables, underscores the critical importance of data quality, integrity, and relevance within modeling endeavors. Any discrepancies, inaccuracies, or biases inherent within data sources, collection methodologies, or analytical techniques may propagate errors, biases, and uncertainties throughout the modeling process, compromising model validity, reliability, and applicability. Consequently, prioritizing data sensitivity mandates implementing rigorous data validation, verification, and quality assurance protocols, fostering transparent documentation, and fostering interdisciplinary collaborations to enhance data accuracy, completeness, and relevance. Moreover, embracing data sensitivity considerations facilitates informed decision-making, policy formulation, and stakeholder engagement, ensuring alignment with epidemiological realities, societal needs, and public health priorities while mitigating potential risks, limitations, and challenges associated with data-driven modeling endeavors.
Case Studies: Real-world Applications of NetLogo in Public Health
Delving into tangible case studies illuminates the pivotal role of NetLogo in addressing critical public health challenges, offering invaluable insights and recommendations for future research and applications. By spotlighting specific instances where NetLogo's computational prowess and analytical capabilities have been instrumental, stakeholders can glean practical lessons, innovative methodologies, and evidence-based strategies that resonate with real-world complexities. These illustrative examples not only showcase the versatility and adaptability of NetLogo across diverse epidemiological scenarios but also foster collaborative learning, informed decision-making, and transformative solutions within the public health landscape. In essence, examining case studies encapsulates NetLogo's impact, efficacy, and potential, serving as a catalyst for advancements, fostering resilience, and shaping a proactive paradigm that prioritizes innovation, collaboration, and excellence in addressing global health challenges.
Infectious Disease Modeling
1. COVID-19 Pandemic Analysis:
In the context of the COVID-19 pandemic, leveraging NetLogo for modeling has proven instrumental in elucidating transmission dynamics, assessing the effectiveness of various mitigation strategies, and informing evidence-based public health policymaking at local, national, and global scales. By simulating diverse scenarios, including population interactions, mobility patterns, vaccination campaigns, and behavioral dynamics, NetLogo facilitates a nuanced understanding of epidemic trajectories, potential outcomes, and intervention impacts. Furthermore, integrating real-time data, epidemiological insights, and contextual variables enhances model fidelity, fostering informed decision-making, resource allocation, and stakeholder engagement. However, navigating the complexities of COVID-19 modeling within NetLogo mandates continuous refinement, validation, and adaptation to address evolving epidemiological dynamics, emerging variants, and multifaceted public health challenges effectively.
2. Vector-Borne Diseases:
NetLogo's application in modeling vector-borne diseases, encompassing malaria, dengue, Zika, and other endemic pathogens, offers invaluable insights into transmission risks, vector control measures, community interventions, and environmental determinants shaping disease prevalence. By simulating intricate interactions between vectors, hosts, environmental factors, and human behaviors, this platform facilitates the exploration of diverse scenarios, enabling stakeholders to assess intervention strategies, optimize resource allocation, and prioritize high-risk populations effectively. Moreover, integrating spatial analyses, climate data, and community dynamics enhances model robustness, fostering a comprehensive understanding of vector-borne disease epidemiology, transmission dynamics, and public health implications. Nonetheless, harnessing NetLogo for vector-borne disease modeling necessitates addressing spatial-temporal complexities, ecological interactions, and interdisciplinary collaborations to ensure accuracy, relevance, and applicability in guiding surveillance, prevention, and control efforts across diverse epidemiological contexts and geographic regions.
Conclusion
Epidemiological modeling stands firm as an indispensable cornerstone within the realm of public health research and practice, serving as a catalyst for informed decision-making and effective disease risk mitigation strategies. The integration of NetLogo into health sciences assignments presents a transformative paradigm, unlocking invaluable opportunities for students, educators, and researchers to delve deeper into complex epidemiological phenomena. By cultivating a nuanced understanding of NetLogo's capabilities, limitations, and diverse applications, stakeholders can strategically leverage its computational prowess to advance public health initiatives. This synergy not only fosters collaborative learning and research but also empowers individuals and organizations to devise evidence-based interventions, policies, and strategies that resonate with real-world complexities. Consequently, harnessing the full potential of NetLogo within the context of epidemiological modeling holds the promise of fostering innovative solutions, enhancing population health outcomes, and shaping a resilient, adaptive, and proactive public health landscape for generations to come.